<TLDR> Rendered.ai joined the Open Geospatial Consortium to contribute to standard processes and patterns for driving more explainability, findability, and reproducibility in tools and techniques for computer vision applied to geospatial problems.
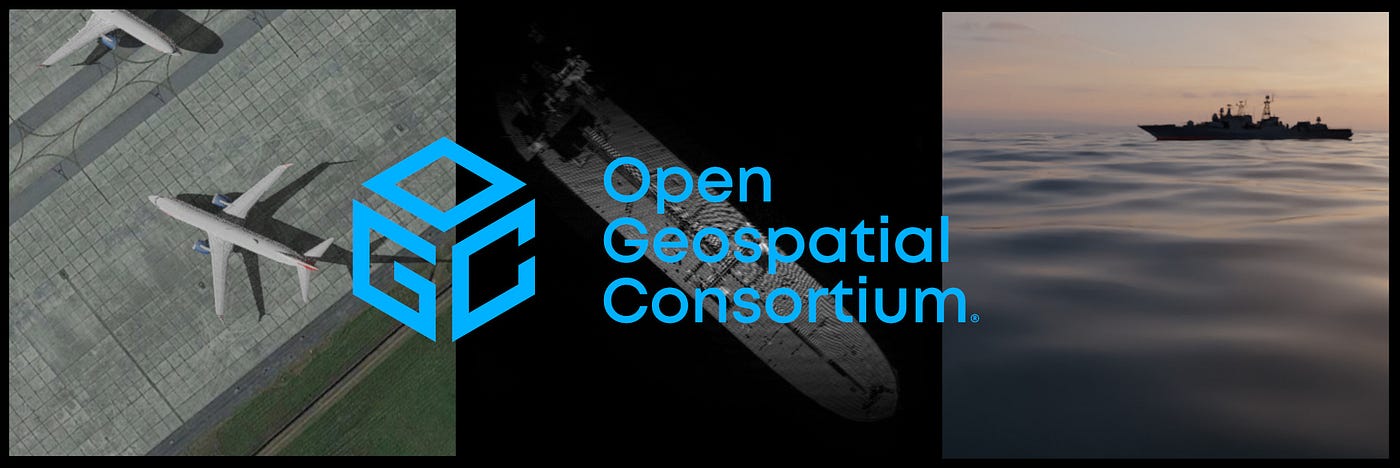
This week, I participated in a virtual event that is one of a series that has demonstrably impacted how users share, consume, and generate value from geospatial data and processes. The Open Geospatial Consortium’s Testbed program has run for approximately 25 years and has been a vehicle for collaboration between academia, government, and commercial industry. In fact, the first OGC testbed I participated in was a CAD-GIS-BIM integration event when I was starting out at Autodesk. That event was an important inflection point for raising awareness, if not setting standards, that the BIM and GIS worlds were going to need to work together. In the following years, I had the fortune to be able to materially contribute to that collaboration and some of the relationships and concepts that were established in that early event certainly influenced my ability to make an impact.
Fast forward to 2023 and multiple factors are driving the increased importance of the collaboration, reproducibility, and knowledge dissemination that are facilitated by organizations such as OGC. Late last year, we decided to become a small business member of OGC. We have been selective about our corporate partnerships and organization memberships and we believe that OGC is currently at a unique juncture to be able to facilitate technology improvement over the next few years that has potential to address critical issues confronting our society. We would like to help.
Three drivers for increase in standardized GeoAI
Three major drivers that I see which will increase the importance of OGC activity relevant to Rendered.ai include:
·The need to transform exponentially increasing amounts of geodata collection of the Earth around us into knowledge about the systems around us from the scale of classrooms to ecosystems
· Challenges to international safety and security from both increased geopolitical friction and human-caused ecological change driving the need for faster and more complex analytics
· Proliferation of edge sensors and processing which will be required to enable autonomy of everything from farming equipment to enabling aging populations to function in changing economic systems
Common issues when sourcing real datasets
At Rendered.ai, we build a platform as a service for generating synthetic computer vision (CV) data to help reduce the challenges of using real sensor data to train AI. Computer vision content from satellite imagery to radar and lidar has the potential to be used to help address every geospatially related problem today. Watching the testbed reports this week, I saw multiple intersections between what we do at Rendered.ai and the work that is being initiated by the participants in OGC Testbed 18. For example, the section on training data standards discussed problems that we hear from customers every day:
· Sourcing the right data to address a specific problem can be difficult or impossible
· Existing datasets available in open repositories or the commercial market are incomplete
· Many solutions require CV data across multiple domains
· Data labeling of real datasets is imperfect and, often, incomplete or inaccurate
· There are few standards for describing what data diversity, annotation, or other metadata should be in a dataset to address specific problems
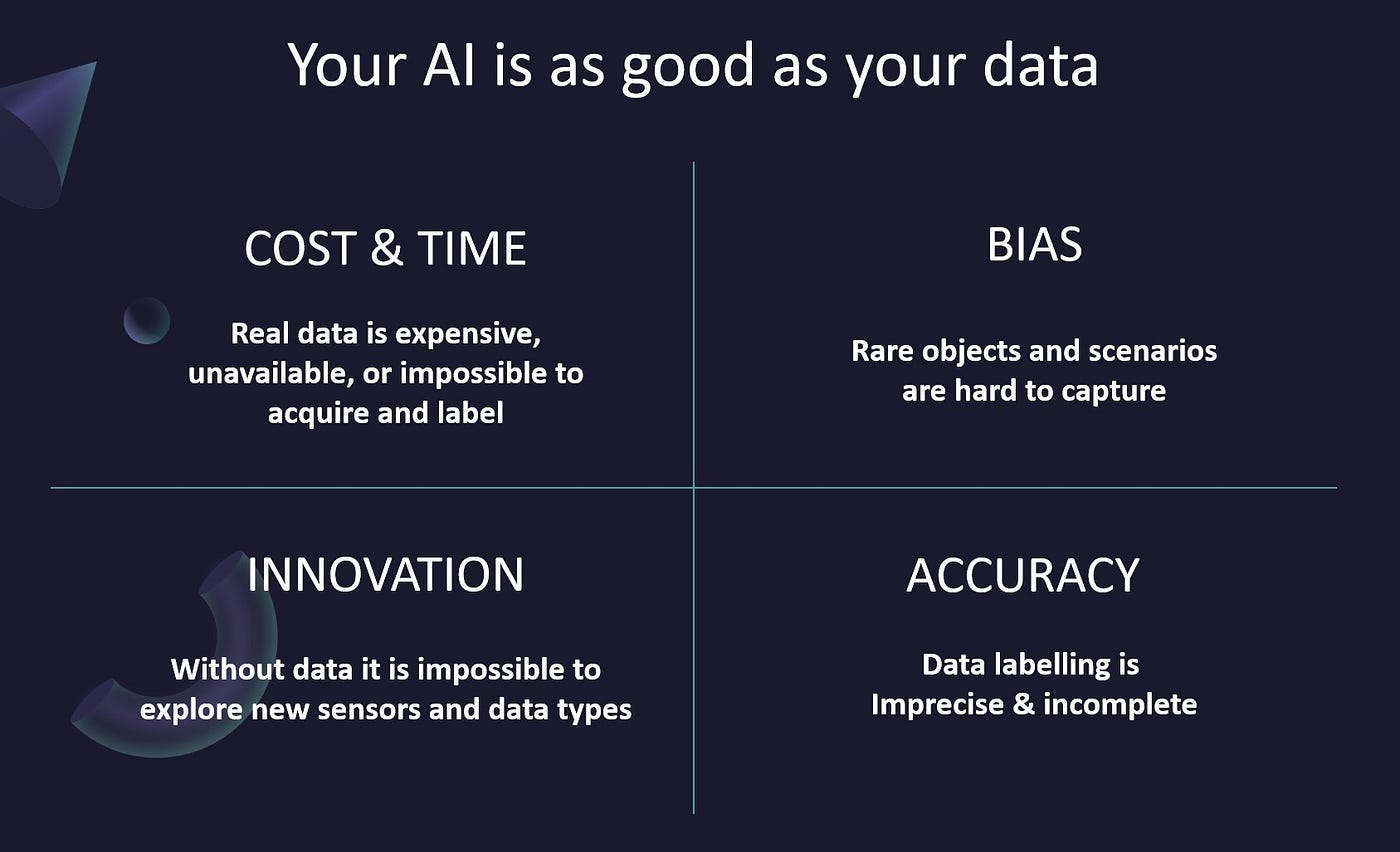
The opportunity of Synthetic Data for GeoAI
At Rendered.ai, hearing these issues excites us because synthetic data has the potential to be used to help mitigate each of these problems. Synthetic data is engineered, intentionally designed data that replicates the qualities of real data for purposes of training and validating AI, while allowing the data scientist or CV engineer to intentionally control for variables that will reduce traditional training issues such as poor diversity in training data, lack of adequate quantities, and unexpected failure caused by issues such as inaccurate labeling.
Imagine if it were possible to produce infinite amounts of the world’s most valuable resource, cheaply and quickly. What dramatic economic transformations and opportunities would result?
This is a reality today. It is called synthetic data.
Synthetic data has the potential to transform data from being an inhibitor to being an enabler. I’m excited to see the content in upcoming Testbed events and hope to be able to contribute more directly as we get involved through our membership. Some of the ways that we can see synthetic data and our platform contributing to the CV community around OGC include:
· Supporting the emergence of standards for training dataset composition — including metadata, labeling, and geoinformation — by encoding them in synthetic data channels that help establish reference datasets for use by the community
· Offering open-source frameworks for building common patterns of generating standards-based training data
· Creating datasets for a wide diversity of sensor types, platforms, and scenarios
· Generating completely open and shareable datasets for experimentation, testing, and validation
As we’ve seen in other parts of the geospatial industry, it’s conceivable that standards around training data for AI become not just recommended, but actually mandated by government agencies and parts of industry. We’ve seen this coming at Rendered.ai as the limitations of real data have significant impact on the quality of trained AI, which is becoming ubiquitous in our society. Synthetic data has been shown to reduce some of those issues and even has the potential to become a required technique for reducing bias and making AI more explainable… in the geospatial computer vision world and beyond!
Moving forward, we hope to contribute more directly to OGC and broader geospatial community projects. Most of our customers, such as Orbital Insight, BigBear.AI, and Faculty are in the geospatial and remote sensing analytics industry, often as direct customers to critical government agencies… including agencies who sponsored this Testbed event!
Get in touch or try synthetic data out!
If you’re interested in finding out more about how synthetic data can help with your GeoAI training needs, please contact us.
You can even try out our platform by submitting this survey.