The Platform for Generating Low-cost Physically-accurate Data, helping Overcome Bias and Powering Innovation in AI and ML
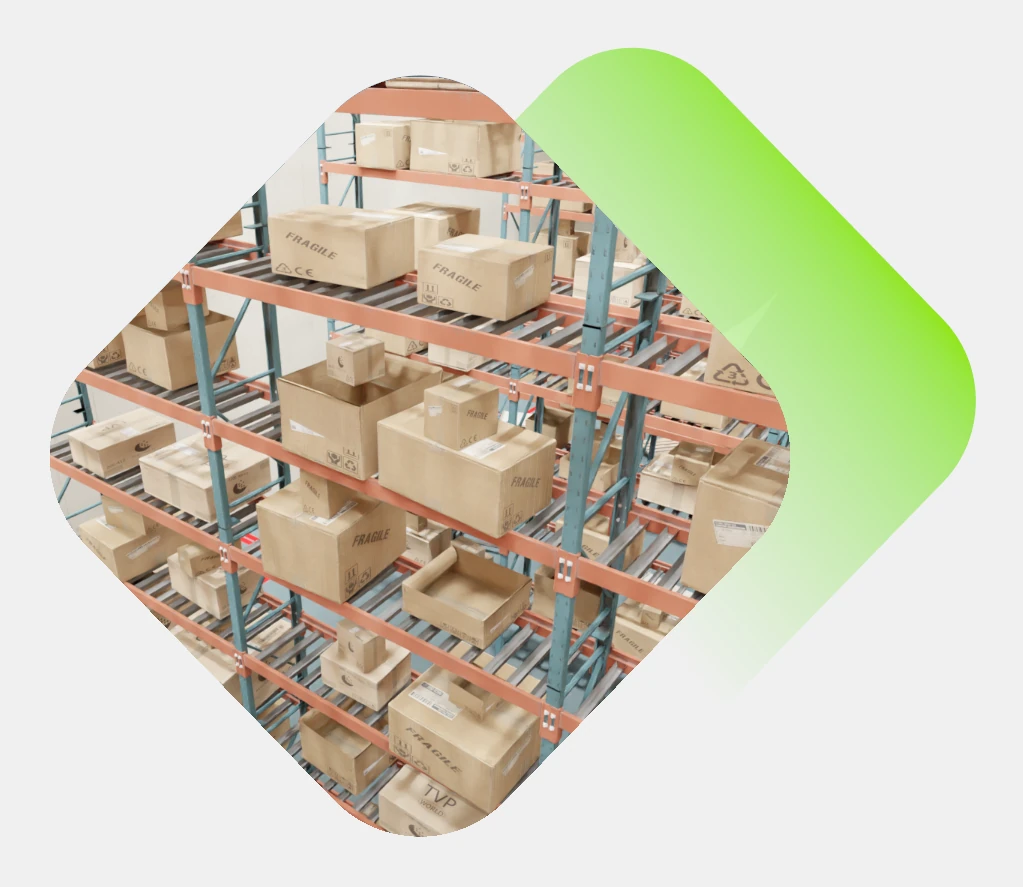
Rendered.ai is the Platform for Generating Synthetic Computer Vision Datasets for Training Artificial Intelligence and Machine Learning Systems
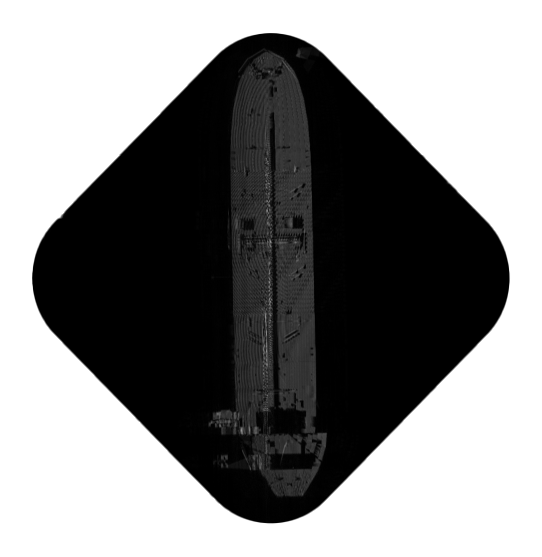
Capture Rare Events & Edge Cases
Reproduce rare events and edge case scenarios that are difficult to capture and record real sensor data.
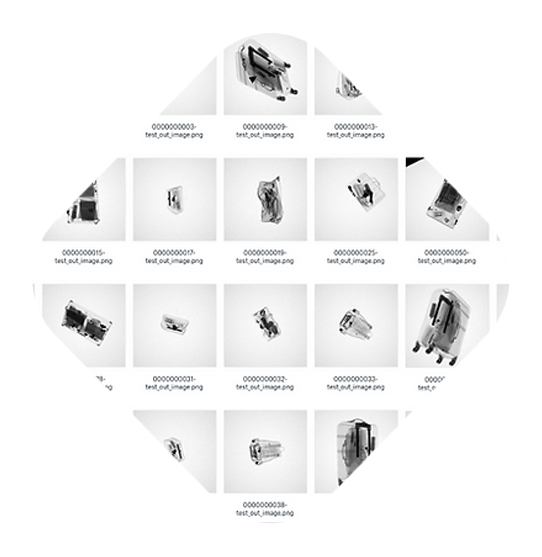
Acquire Data to Innovate
Generate data that is impossible or difficult to acquire because sensors don’t exist or acquisition cost is prohibitive.
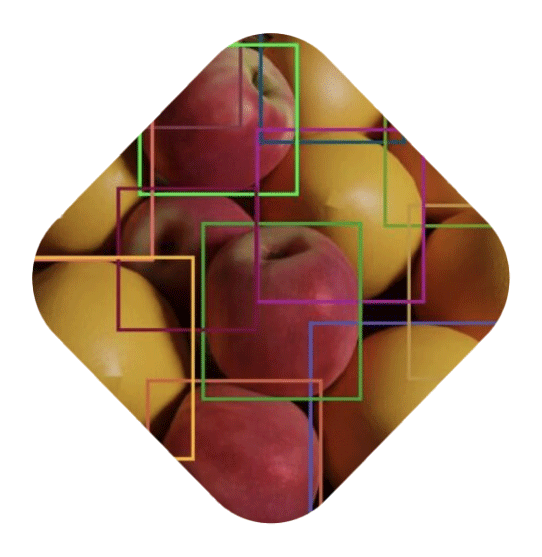
Overcome Data Labeling Challenges
Generate 100% accurately labeled data, including sensor data that humans have difficulty reading or identifying.
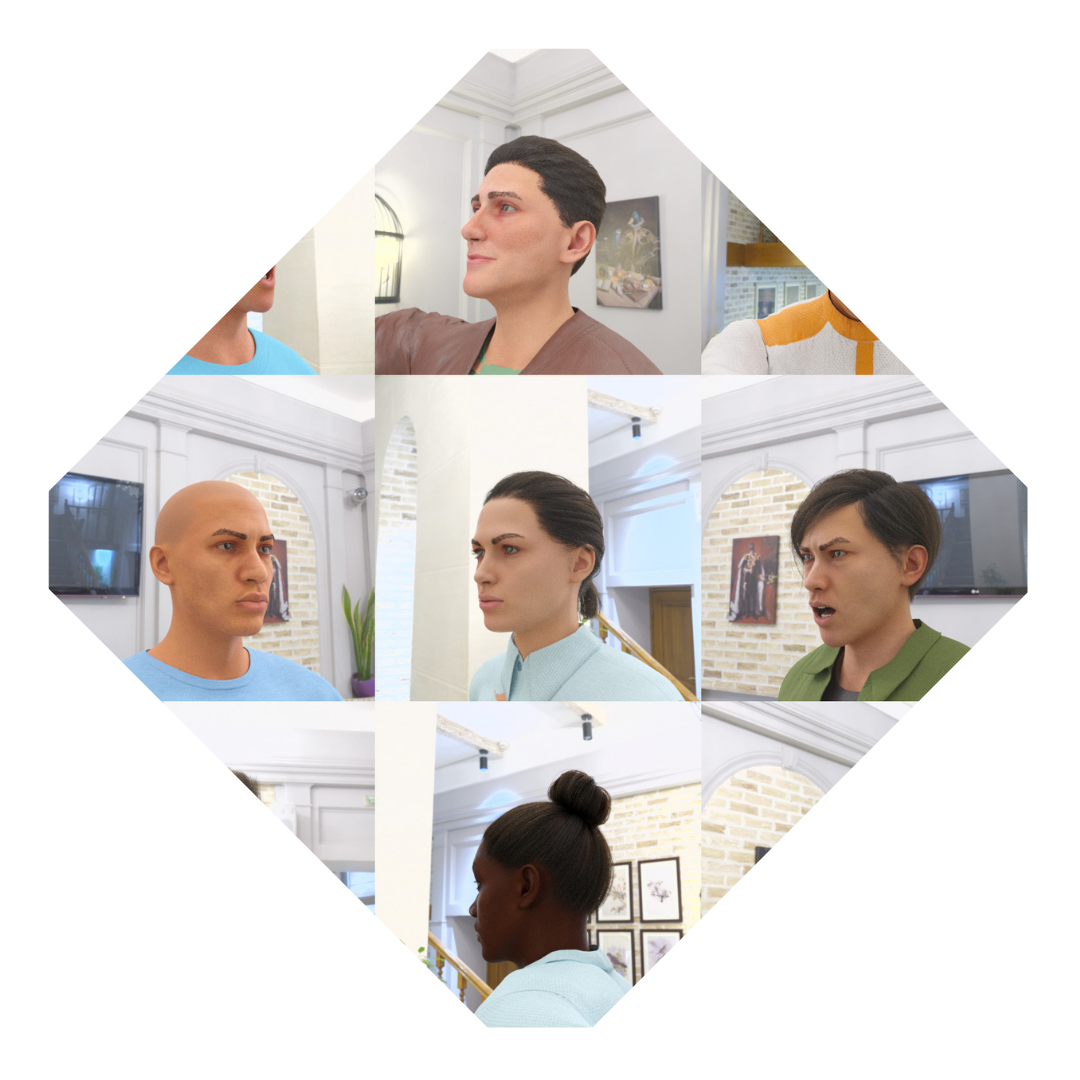
Reproduce Restricted or High Risk Data
Simulate datasets of high risk scenarios, datasets that would be restricted by security, health care, or privacy laws.
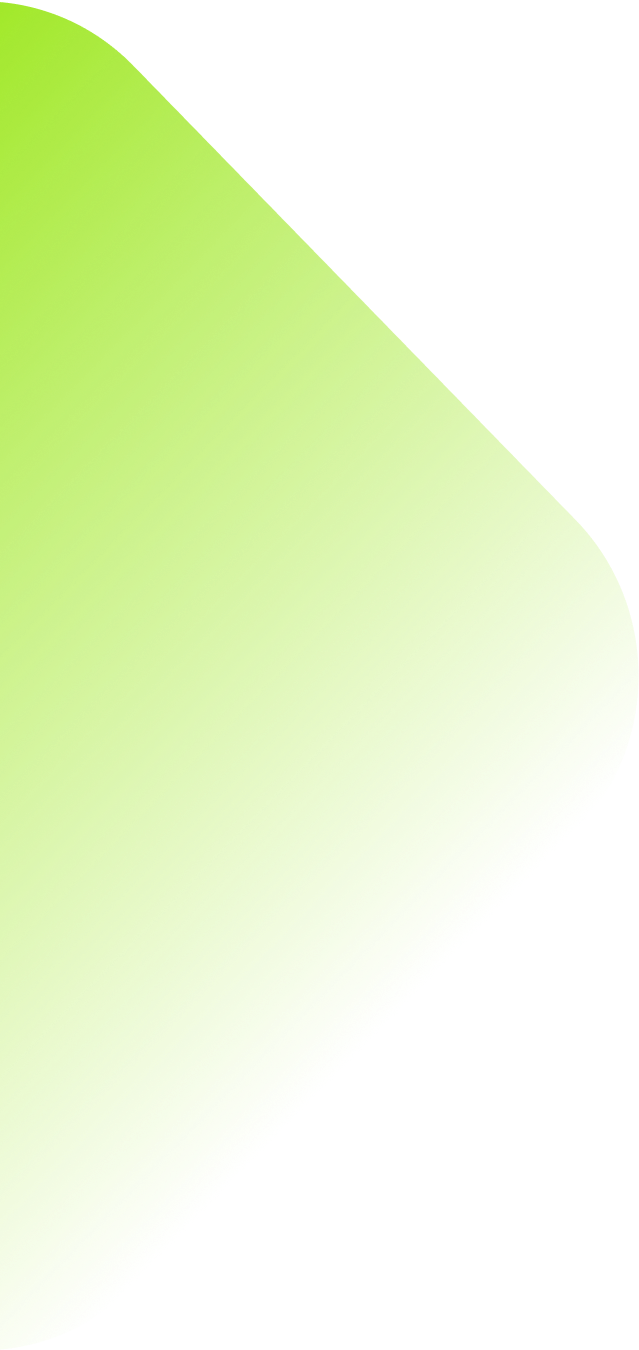
“By 2024, 60% of data used for AI and data analytics projects will be synthetic, and by 2030, synthetic data will have completely overtaken real data in AI models.”
- Gartner, September 2021
Spending Too Much Time and Money and Not Getting the Data You Need?
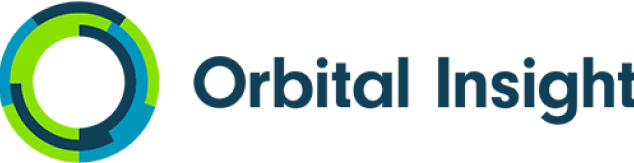
“Working with Rendered, we were able to use synthetic data to significantly improve computer vision algorithm performance for detecting economically important objects in satellite imagery. AP scores improved across the board for identifying rare and unusual objects when combining synthetic images with actual satellite imagery compared with using real images alone. The objects in our study have been historically difficult to identify using automated algorithms but represent high value information for monitoring and reporting.”
“We saw that synthetic data is a critical capability for computer vision algorithm development and for optimizing detection performance to extract vital information from real sensor-based data.”
James Crawford, Orbital Insight Founder, Chairman, CTO
Our Partners
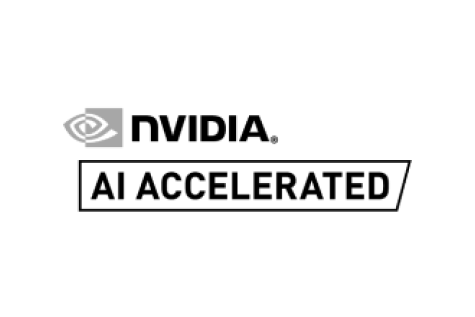
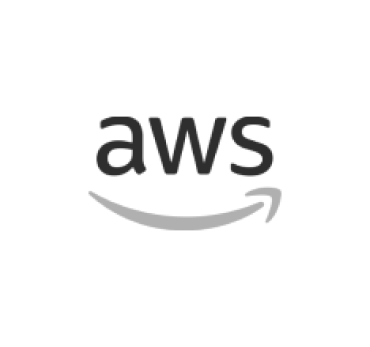
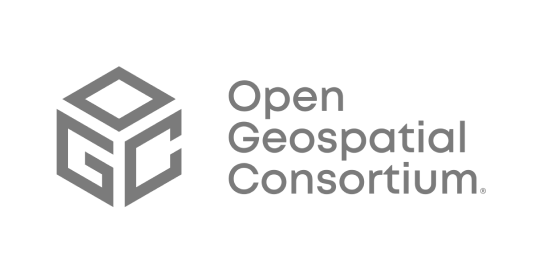

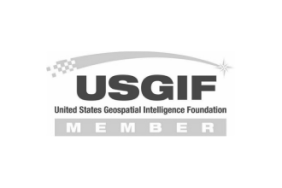
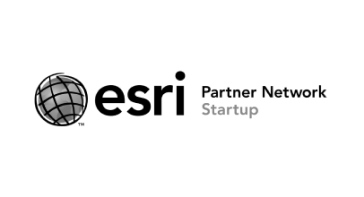
Featured Articles
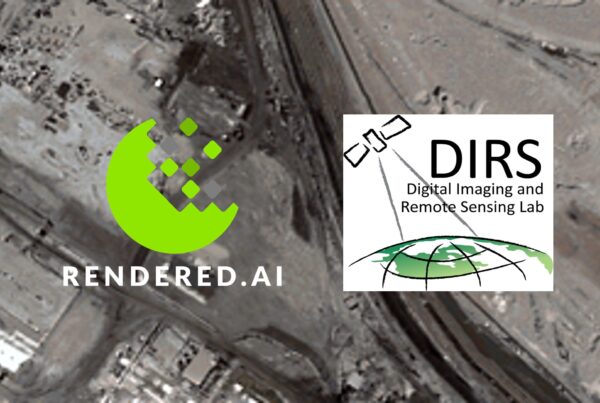