A Cutting-Edge Platform Designed to Enable Innovation in Computer Vision with Synthetic Data
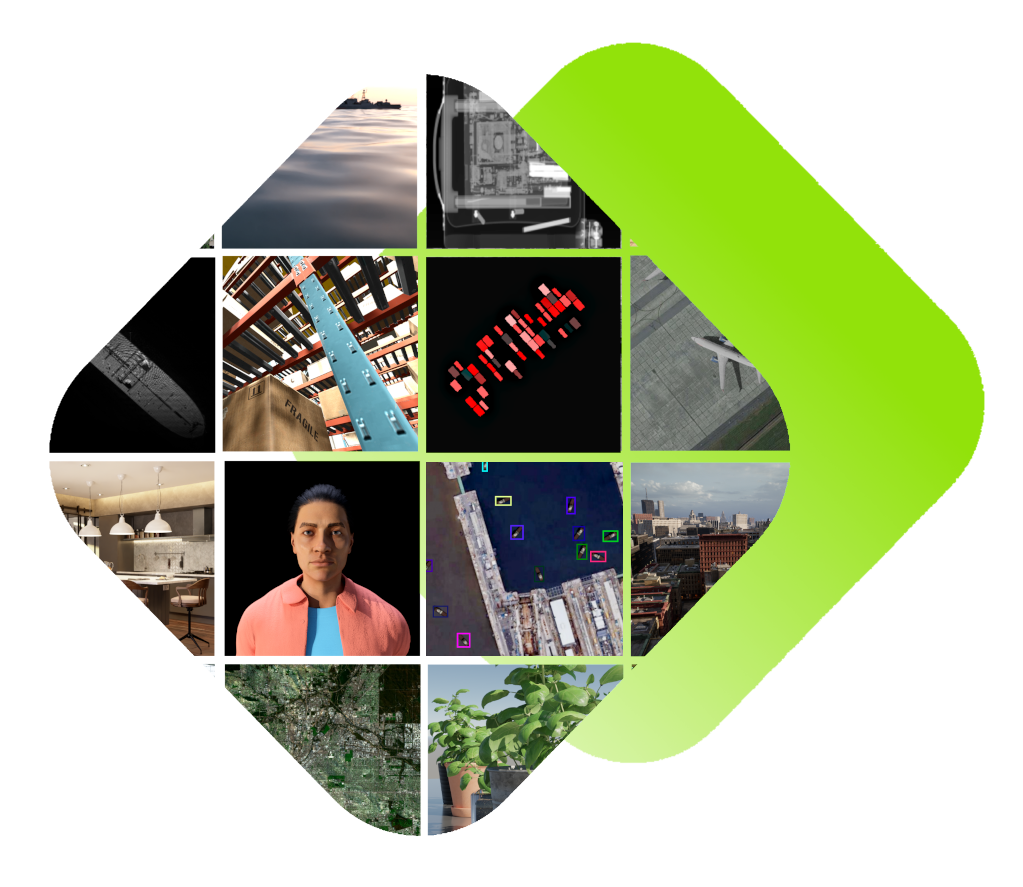
Defense and Intelligence
The defense and intelligence sectors face unique obstacles in acquiring datasets for objects and scenarios that are rare and sensitive. The nature of defense operations and classified information requires high reliability that training data can be used to create accurate detection systems and that it can be produced in a rapid turnaround time to meet changing conditions in the field. Obtaining data that represents never-before-seen objects or hazardous events and conditions is also challenging.
By making it easy for computer vision teams to simulate complex defense and intelligence scenarios along with data capture according to the specifications of real space, aerial, and ground systems, Rendered.ai enables customers to simulate data with the diversity and characteristics required by specialist analysts and data science teams.
Synthetic data generated with Rendered.ai empowers the training and validation of AI models for many applications including threat detection, situational awareness, and condition assessment.
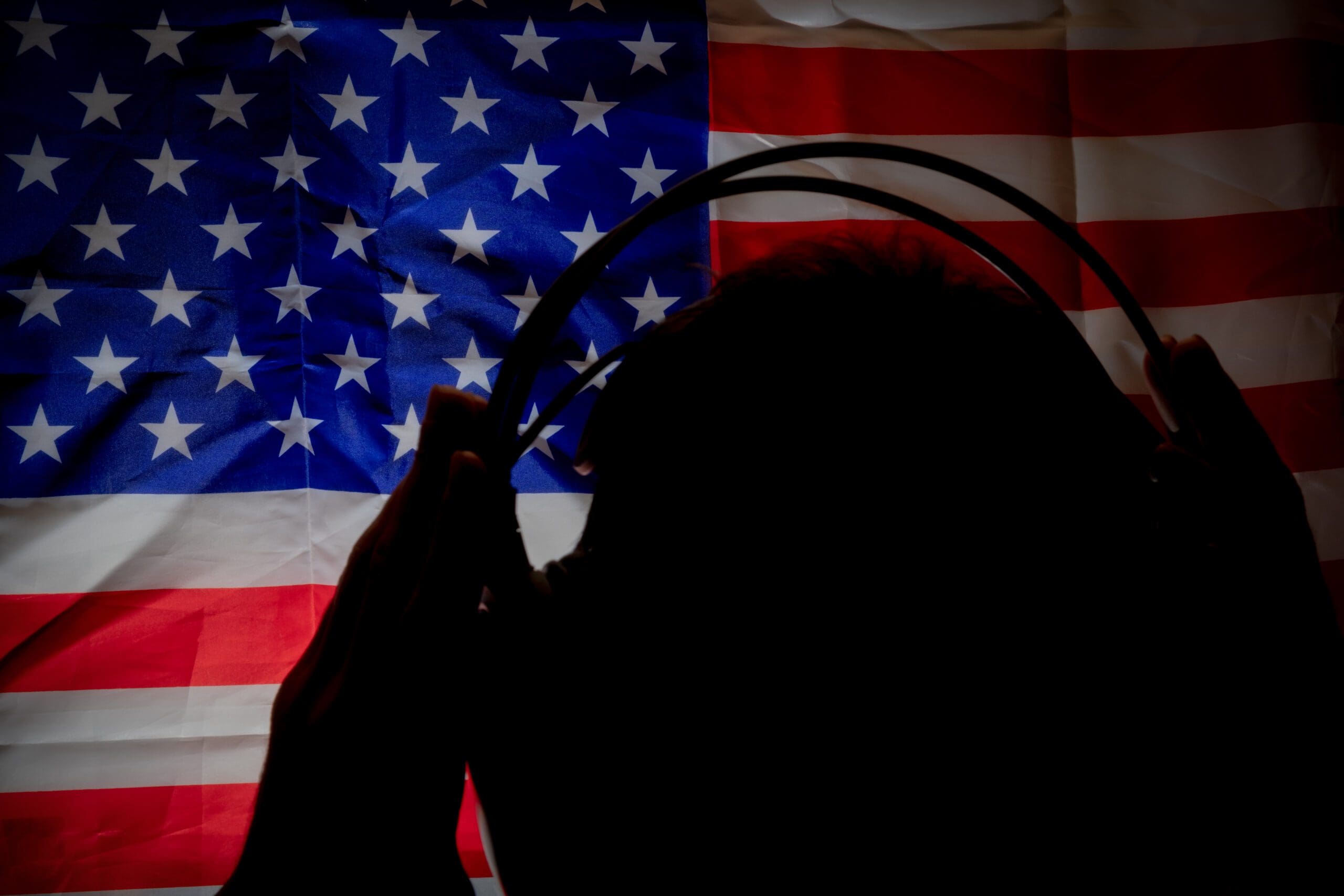
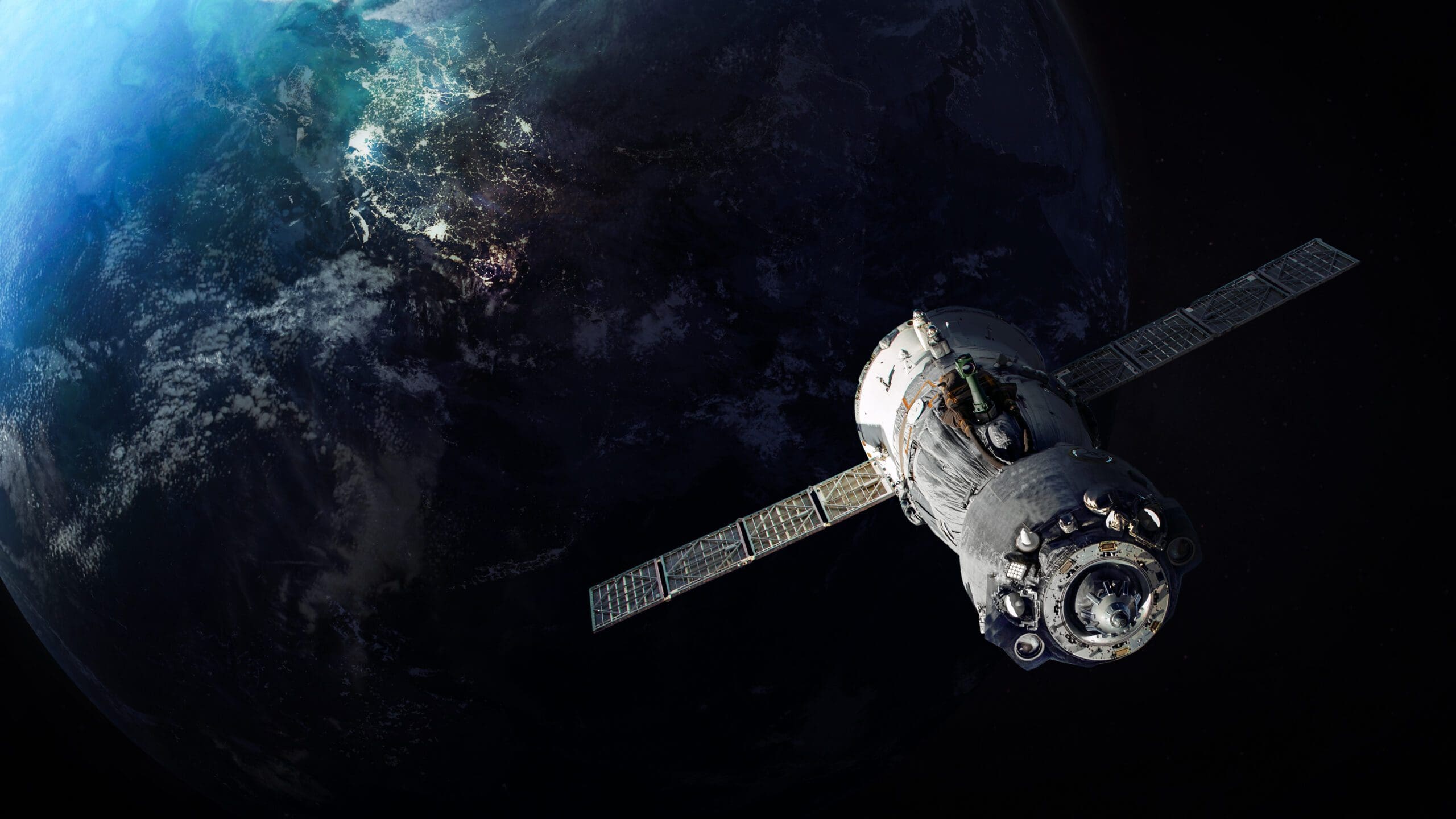
Earth Observation
Earth Observation, a vital field that involves the gathering and analysis of data from satellites and other remote sensing technologies, encounters unique obstacles in training algorithms to extract information and features from diverse imagery sources.
The acquisition of accurately labeled data that is up-to-date and which meets a specific computer vision problem with minimal bias is essential for a wide range of Earth Observation applications, such as ecological monitoring, disaster management, and security monitoring. In ability to access new data types, security limitations, and financial constraints hinder AI/ML training in many Earth Observations use cases. To surmount these hurdles, Rendered.ai offers an innovative solution through its state-of-the-art synthetic data generation capabilities.
By simulating Earth Observation data, including a broad selection of physically accurate sensor modalities such as Electro-optical, SAR, hyperspectral, and multispectral imagery, Rendered.ai empowers customers to train algorithms to detect and extract information with data that would otherwise be cost prohibitive or completely unavailable because of industry constraints.
Transportation
Computer vision applications abound throughout the transportation industry. From road condition assessment to rail safety monitoring to autonomy, transportation relies on rapid detection of issues and conditions that are used to make informed decisions and ensure the safety and efficiency of operations.
In recent years, the integration of synthetic computer vision data has emerged as a valuable tool that mimics real-world scenarios and can be used to train and validate computer vision algorithms across a wide variety of transportation industry applications from the manufacturing line to the field.
Gathering large-scale, high-quality datasets for transportation-related tasks can be challenging, especially when it comes to rare or hazardous situations. Synthetic data can be used to supplement existing datasets by generating additional instances of specific scenarios or capturing edge cases that are difficult to encounter in reality.
By combining real-world and synthetic data from Rendered.ai, transportation organizations can improve the robustness and generalization capabilities of their computer vision algorithms, enhancing the overall performance of applications such as object detection, asset management, and passenger monitoring.
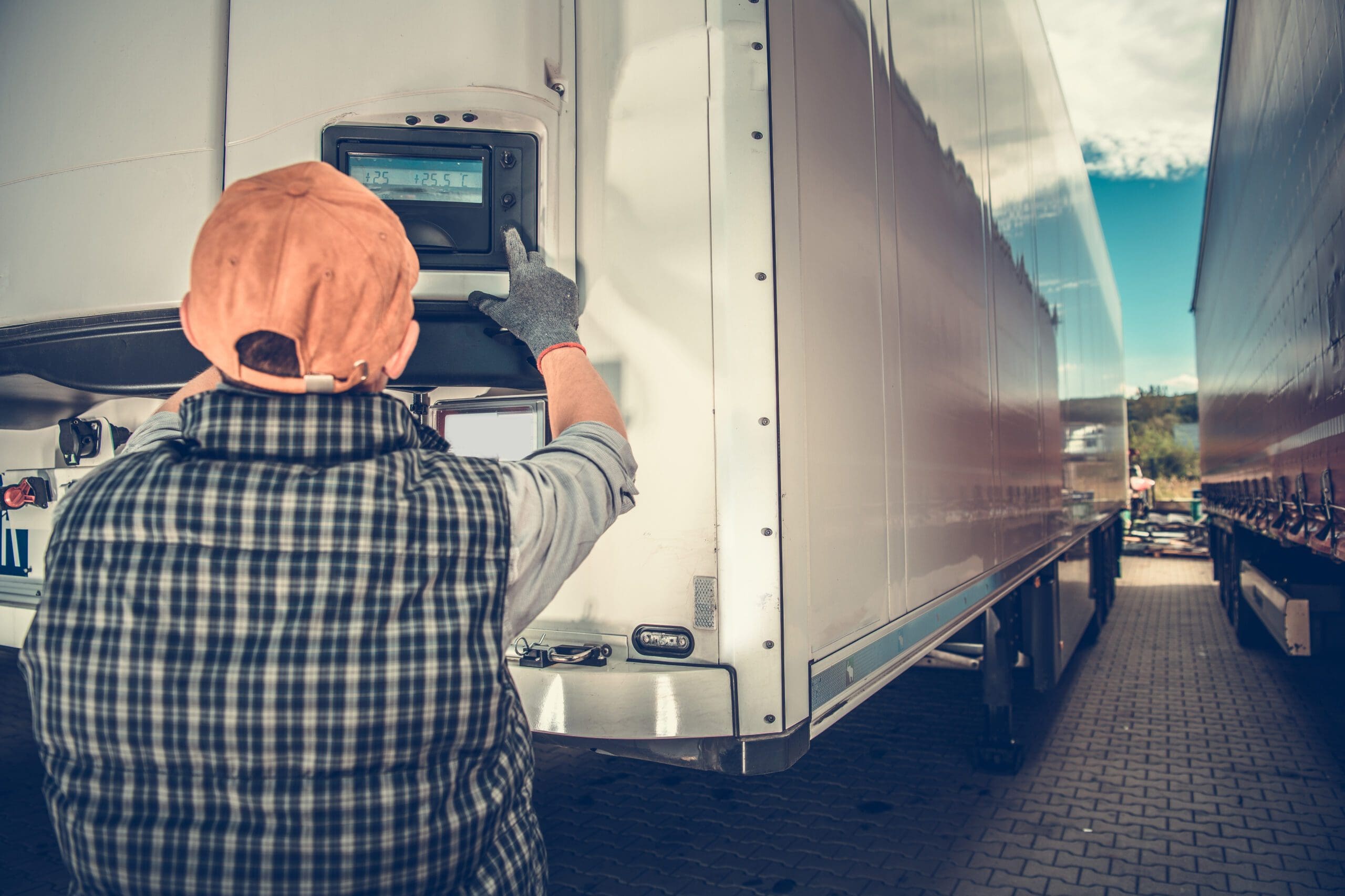
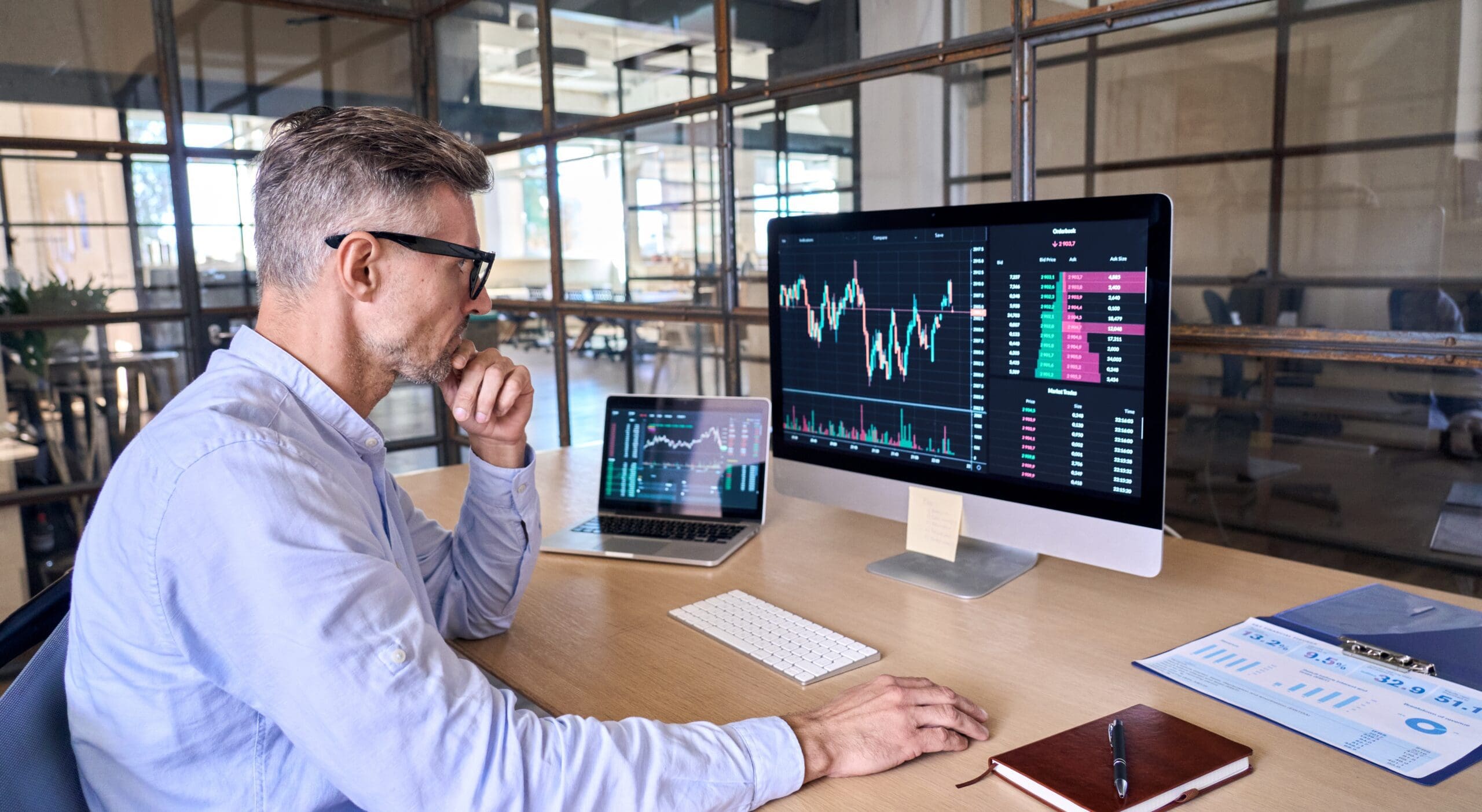
Insurance
Computer vision applications in the insurance industry can speed identification of damage and accelerate coverage payment, accurately identify asset conditions in the field, and can also be used to influencing health-based decision systems. Reduction of bias, access to diverse training data, and eliminating the use of high risk private data for training algorithms are critical steps to applying AI to insurance industry applications.
By using synthetic data, insurance companies can generate a vast range of simulated damage scenarios, allowing algorithms to learn and accurately identify different types and degrees of damage.
Similarly, in infrastructure insurance, synthetic data can be employed to train algorithms to detect and assess the condition of bridges, buildings, or other critical assets, helping insurers identify potential risks and improve risk management strategies.
Using a robust platform such as Rendered.ai to design data to address challenges related to bias, data diversity, and privacy, synthetic data can revolutionize the way insurance companies automate their business models and provide more efficient and accurate services to their policyholders.
Manufacturing and Logistics
In Manufacturing and Logistics, loss reduction, inventory, and safety of goods and people are essential to the smooth functioning of the industry. Computer vision is already broadly applied to monitor, track, and count people, parts, and defects throughout the supply chain that leads to the goods and food that we consume every day.
Generating large, diverse datasets can be challenging and the speed of deploying AI and ML systems can lead to massive savings or lost opportunities. By leveraging synthetic machine vision data from the Rendered.ai platform, customers in the manufacturing and logistics industry can create extensive and diverse datasets that can be used to train models for monitoring, inspection, and tracking.
Synthetic data can be created to simulate assembly lines, warehouses, or transportation routes, providing training data for algorithms to accurately detect, identify, and analyze people, parts, and defects in real-time. With Rendered.ai, synthetic data offers a cost-effective and scalable solution for generating massive amounts of diverse data, reducing the need for and expense of manual data collection.
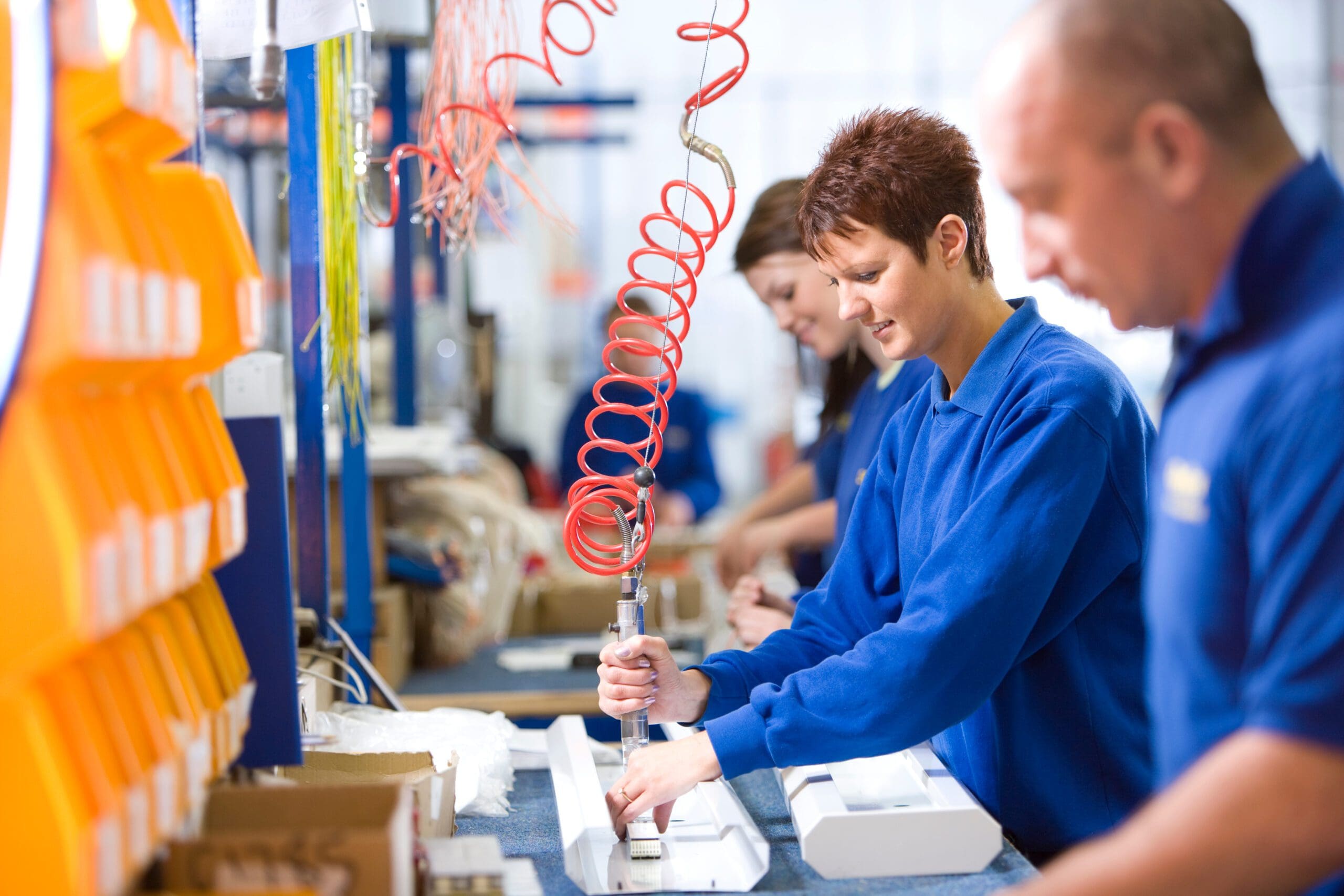
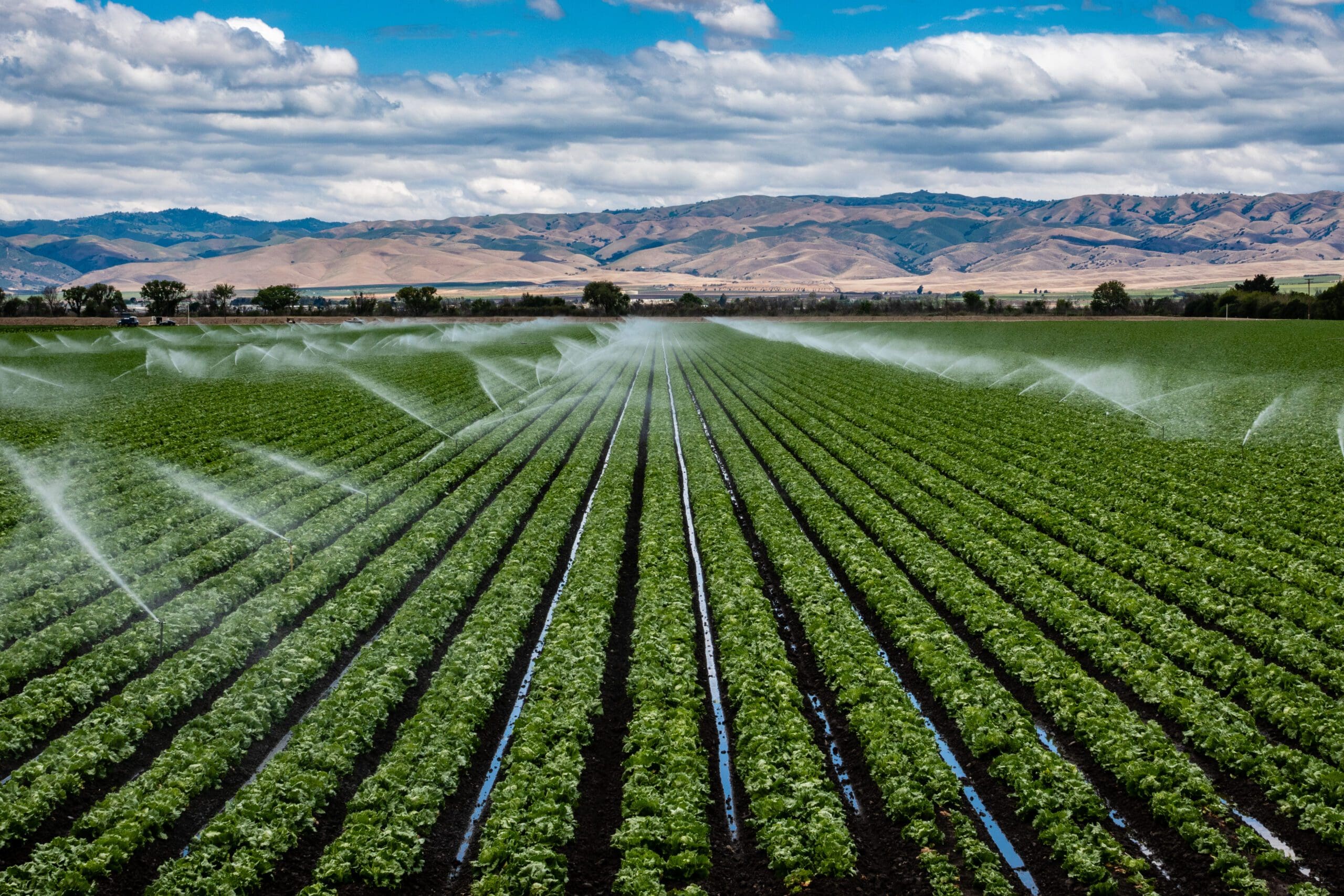
Agriculture
Whether measuring ripeness and acreage in satellite imagery or identifying blight in a photo on a mobile app, the agriculture industry is beginning to rely on computer vision to detect problems, decrease waste, and feed the planet.
By generating synthetic data that represents different crop types, growth stages, and environmental factors, computer vision models can be trained to accurately identify and analyze agricultural parameters, such as crop health, pest infestations, or yield predictions. Better data enables farmers and agricultural organizations to make informed decisions, optimize resource allocation, and implement precision farming techniques, ultimately leading to increased productivity, reduced waste, and improved food production capabilities to meet the growing global demand.
Synthetic data can also be used in the agriculture industry to train computer vision models for weed detection, helping farmers identify and target invasive plants more effectively. Additionally, synthetic data can assist in the development of computer vision systems that can identify and categorize soil types, enabling precision soil analysis and fertilization practices.
The Rendered.ai platform enables customers to design the synthetic data that they need to address a broad variety of computer vision applications across the agriculture industry.