What are the Benefits of Using Synthetic Data to Train Computer Vision Algorithms?
Quality data will always be a business requirement in the dynamic landscape of AI and ML development. Real sensor data is crucial for training algorithms, but acquiring and using real data effectively comes with its own challenges. With cost and time constraints to accuracy and consumer privacy issues, relying solely on real data can lead to schedule delays, higher training costs, and even the inability to train algorithms adequately. However, a powerful solution has emerged: synthetic data. By integrating synthetic data into training workflows, developers can tackle the challenge of using only real data, enabling more efficient and innovative AI/ML solutions.
The Challenges of Real Data
- Cost & Time: Acquiring and processing real data can be expensive and time-consuming, hindering development.
- Data Availability: In many cases, the amount of real data available is insufficient for algorithm training and validation. For some projects, especially when dealing with new sensors or types of data collection, real data may not yet exist, preventing any model development before physical sensors are deployed.
- Bias: All datasets have limited distributions of objects and classifications, leading to detection bias, and these distributions can’t be controlled easily or cost-effectively in real datasets.
- Accuracy: Labelling of real data often suffers from inaccuracies, especially in labeling, which can compromise the effectiveness of training algorithms and complicate dataset integration.
- Privacy and Security: Using some kinds of real-world data raises significant privacy and security concerns, which may limit the ability to share and use data to train algorithms.
Physics-Based Synthetic Data
Synthetic data is engineered data generated by a computer and intended to simulate data collected in the real world. With the rapid emergence of Generative AI and tools for using AI to create imagery and other content, the market is becoming more familiar with synthetic data. However, there is a difference between generating datasets based on previous data instances and simulating physically accurate data. Physics-based synthetic data generation uses techniques such as 3D modeling and mathematical models to create datasets that can be validated and used as if they were actual sensor-collected data.
To learn more about generative synthetic data versus physics-based synthetic data, watch this quick video with Rendered.ai’s CEO, Nathan Kundtz.
Benefits of Using Physics-Based Synthetic Data
- Cost-Efficiency: Synthetic data generation typically costs a fraction of acquiring real sensor data, enabling rapid and cost-effective iteration.
- Customization: Tailoring datasets to specific requirements reduces post-processing efforts and streamlines development.
- Controlled Bias: All datasets have bias. With synthetic data, users can control the distribution of objects and scenarios in their data to add controls for specific training needs.
- Innovation: Accessing synthetic data facilitates experimentation with edge cases and scenarios where real data is scarce or completely unavailable.
- Risk Mitigation: Synthetic data eliminates the risks associated with using sensitive real-world data, ensuring compliance with regulations and safeguarding privacy.
3 Tips for Leveraging Synthetic Data
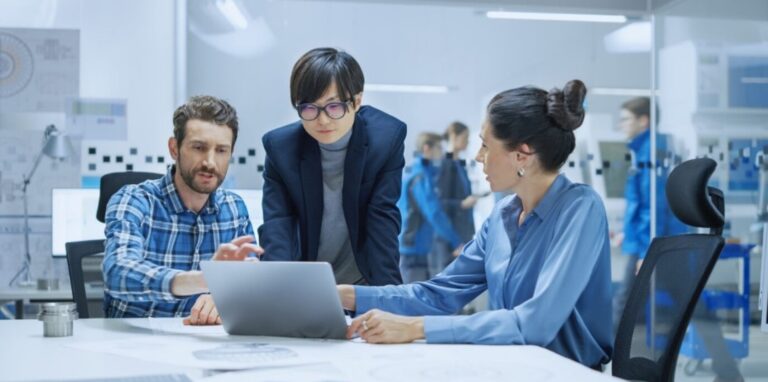
Data engineers and developers actively using synthetic data to train computer vision models today provide the following advice to maximize your advantage:
- Use synthetic data in the development stages of AI and ML systems to significantly reduce costs, increase efficiency, and expand the versatility of your training data library.
- Tap into the combined power of real and synthetic data. Look for data that is accurately labeled to merge datasets more easily and train computer vision algorithms with the best data available for that use case. Accurate labeling also delivers the ability to compare real and synthetic data without confusion.
- Leverage rapid and inexpensive synthetic data generation to continually train AI and ML on new and unusual scenarios where real sensor data may not be available or is too expensive or difficult to acquire for testing.
Unlocking the Potential of Physics-Based Synthetic Data with Rendered.ai
Rendered.ai offers a comprehensive Platform as a Service (PaaS) solution, empowering teams to harness the full potential of synthetic data through:
- A Collaborative Environment: Seamlessly share assets, sensor models, and datasets to enhance team efficiency.
- Cost-Effective Subscriptions: Access unlimited synthetic data generation at a fraction of the cost of acquiring real data.
- Accurate Labeling: Benefit from 100% accurately labeled datasets for reliable model training.
- Dataset Comparison Features: Easily compare real and synthetic datasets for informed decision-making.
- Physically Accurate Rendering: Use validated simulators like RIT’s DIRSIG, NVIDIA Omniverse Replicator, Blender, and more for realistic imagery rendering.
- An Open-Source Framework: Integrate synthetic data generation seamlessly into AI pipelines using a well-documented SDK.
Take the Next Step
Ready to revolutionize your AI and ML development? Try the Rendered.ai PaaS today and experience the power of physics-based synthetic data firsthand.
With synthetic data seamlessly integrated into your workflows, the possibilities for innovation are limitless. Embrace the future of AI development with Rendered.ai and unlock new horizons of efficiency and creativity in computer vision.