We don’t teach data scientists about 3D Graphics — and we shouldn’t. The skillset required to create synthetic datasets fundamentally differs from the skillset required to analyze and use data. With synthetic data improving AI performance, driving down bias, and enabling innovation, a new type of engineer will be essential to the success and competitive advantage of organizations who are adopting AI and ML systems. We call these future heroes ‘Synthetic Data Engineers.’ Synthetic Data Engineers will need to be skilled in some of the most exciting technologies on the market: 3D, game engines, and physics-based simulation — and they will need tools to use these skills in support of Data Scientists.
Learn what skills will be required to be a Synthetic Data Engineer at GEOINT 2022 on April 26 in Aurora, Colorado!
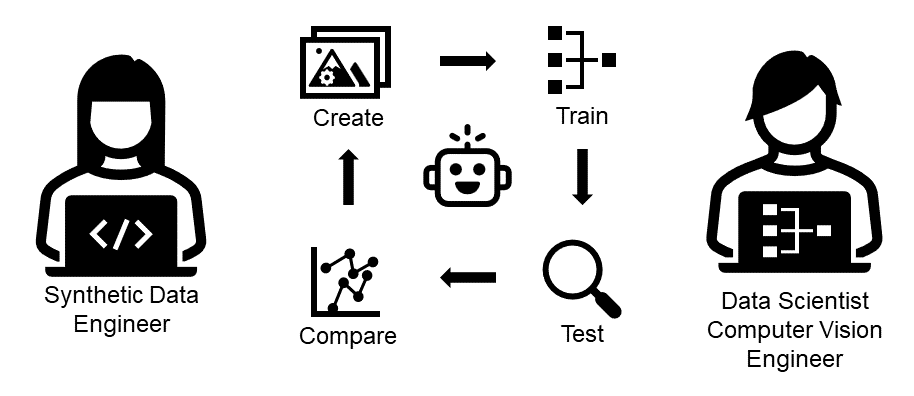
Understanding the problem
If, as Gartner believes, 60% of data used to train AI will be synthetic data by 2024, who is going to be generating all of that data? Today, we find that many companies are starting to hear about synthetic data and are beginning to investigate it, however they often don’t know how to begin creating it.
The first consideration is to look at the type of data being used to train an organization’s AI and ML systems. In our focus area, Computer Vision (CV) data, most customers’ first instinct is to say, “We just need to buy, collect, beg, or borrow more sensor data!” When customers see the cost, schedule, and environmental impact of collecting millions of images, videos, or 3D scans to get a few thousand instances that can be used to train a model, they start to consider options, such as synthetic data.
Synthetic data, for those who may not know, is engineered or fake data produced to specifications determined by real data that is used to train AI. Because synthetic data is designed, it can be created to meet specific training needs, such as reducing bias, rare object detection, or even training for data that doesn’t exist yet.
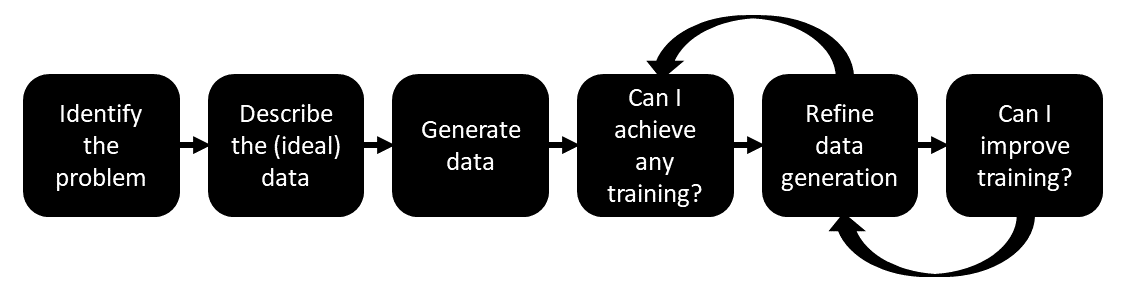
For CV customers*, customers must define the type of training data that is required. This includes understanding file formats, sizes, number of channels and bits-per-channel in imagery datasets, movement characteristics of the imaging platform and much more. Essentially, the customer needs to be able to characterize data collection such that it can be successfully simulated.
The next task becomes defining the world that needs to be imaged or collected in simulated sensor data. CV applications range from identifying rare species of plants and animals in wildlands, to counting military vehicles in urban areas, to detecting problems in factory manufacturing… they are endless.
These requirements are used to define a synthetic data application, which we call a ‘channel.’ In the Rendered.ai PaaS, a user can deploy a channel, create graphs that describe specific dataset configuration, and generate datasets over and over to achieve desired results.
Curious? Sign up for a free Rendered.ai account and generate data for yourself!
Hiring game designers for a data science team?
At this point, many organizations may be scratching their heads thinking something like, “Just how do I simulate images from a camera that hasn’t been designed yet and which will be flying over the Earth on a satellite at 17.500 mph?”
The answer is, there’s tech for that. In the last 10 years, the evolution of 3D hardware and software technologies have reached levels of speed, interactivity, physics-based accuracy, and realistic rendering (picture generation) that has the potential to put simulation technology in the hands of any scientist, engineer, or analyst across most CV domains.
The Synthetic Data Engineer is the experienced professional who can bring together many of these skills and technologies to be able to design and create new types of CV content for training AI. In fact, our expert team is often taking on the role of the Synthetic Data Engineer for our customers.
Some of the specific tools and skills that we have found useful for Synthetic Data Engineering include:
- 3D modeling tools, formats, and specifications
- Animation
- Game engine development
- Geospatial technology and data, GIS tools
- Simulation modeling
- Image manipulation
- Python programming
There are not-so-small corners of the high tech industry that have been incubating Synthetic Data Engineers for a while. We’re seeing highly qualified candidate come from the VFX (visual effects), Defense M&S (Modeling and Simulation), game development, and even the Architecture and Engineering communities.
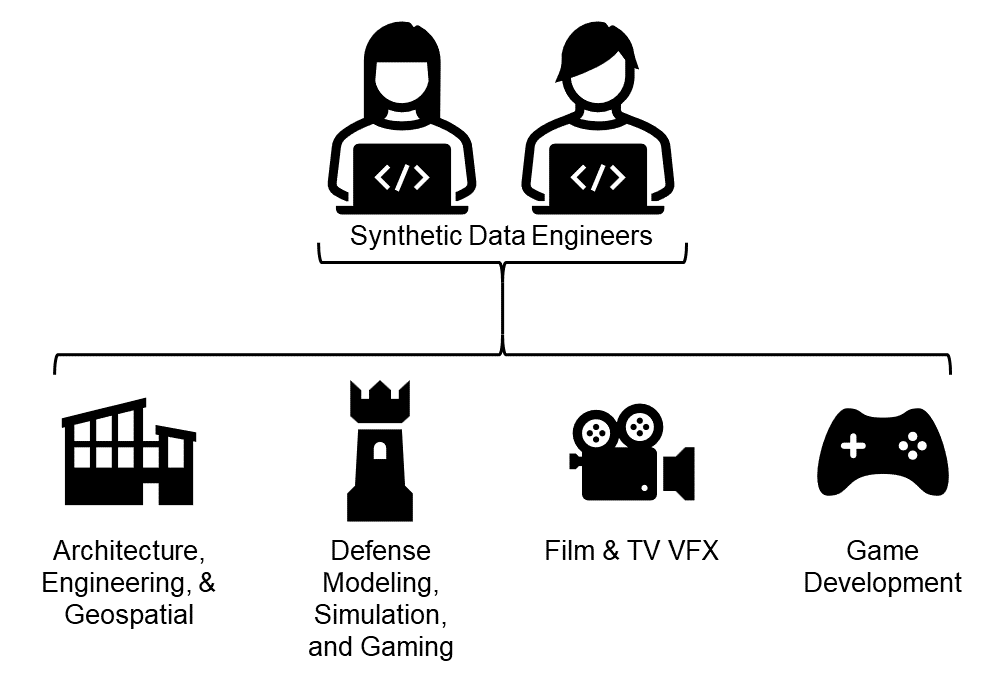
Why isn’t everyone hiring 3D developers to generate Synthetic Data?
It turns out that many CV-oriented organizations have tried to create synthetic data at some point in their AI evolution. If they thought they could hire up a game developer or a VFX artist, generate a few datasets, and then go on with their business, they were likely wrong.
Synthetic data generation tends to be an iterative, evolving capability that requires much more than just the Synthetic Data Engineer’s skills. Organizations also need access to cloud-based job management, user management, dataset curation and search, custom annotation generation, dataset characterization and comparison, and many other tools that enable users to design, generate, analyze, and organize synthetic data. These capabilities essentially are ubiquitous platform needs of anyone generating synthetic data, which is why we built the Rendered.ai PaaS!
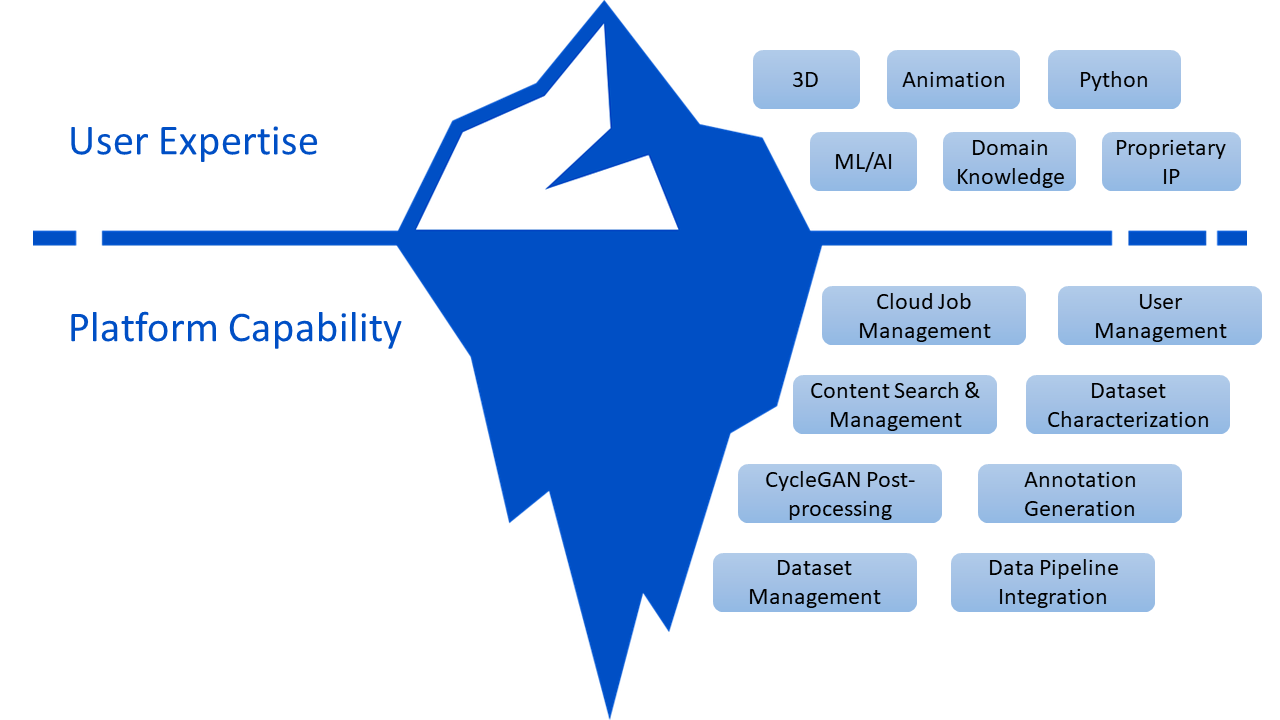
We are already seeing that access to a PaaS for synthetic data generation can expand the opportunity for successful AI adoption in organizations by enabling teams to adapt cutting edge market capabilities in 3D and simulation.
What other skills are helpful for Synthetic Data Engineers?
As a contributor to a team focused on designing high performance AI/ML capability that can enable an organization to compete, innovate, and adapt, the Synthetic Data Engineer needs to be able to work with a variety of peers and stakeholders to have optimum impact. Some of the skills that we also see adding value include:
- Familiarity with ML/AI/DL techniques
- Statistics experience and background
- Familiarity with the sensor domain of the business or organization
- Ability to translate requirements from data scientists, end stakeholders, and data collection experts into code
Learning more
If you’re interested in learning more about synthetic data, Rendered.ai, and some of the skills that are needed to create successful synthetic CV content, check out some of the following links:
- Brief overview video of Rendered.ai
- Learning path on our support site
- A recent webinar overview of our platform
We even open source much of our tools for Synthetic Data Engineers who want to dive in:
- Take a look at our Example channel on GitHub
- Anatools is our open source API for integrating with the PaaS
And don’t forget to sign up and try out Rendered.ai for yourself:
— — — — — — — — — — — — — — — — — — — — — — —
*In the case of text content, NLP training, and other speech and written data, we don’t dabble too much in that space, but there are some great companies out there who are doing so. Take a look at Gretel.ai or Tonic.ai, for example.