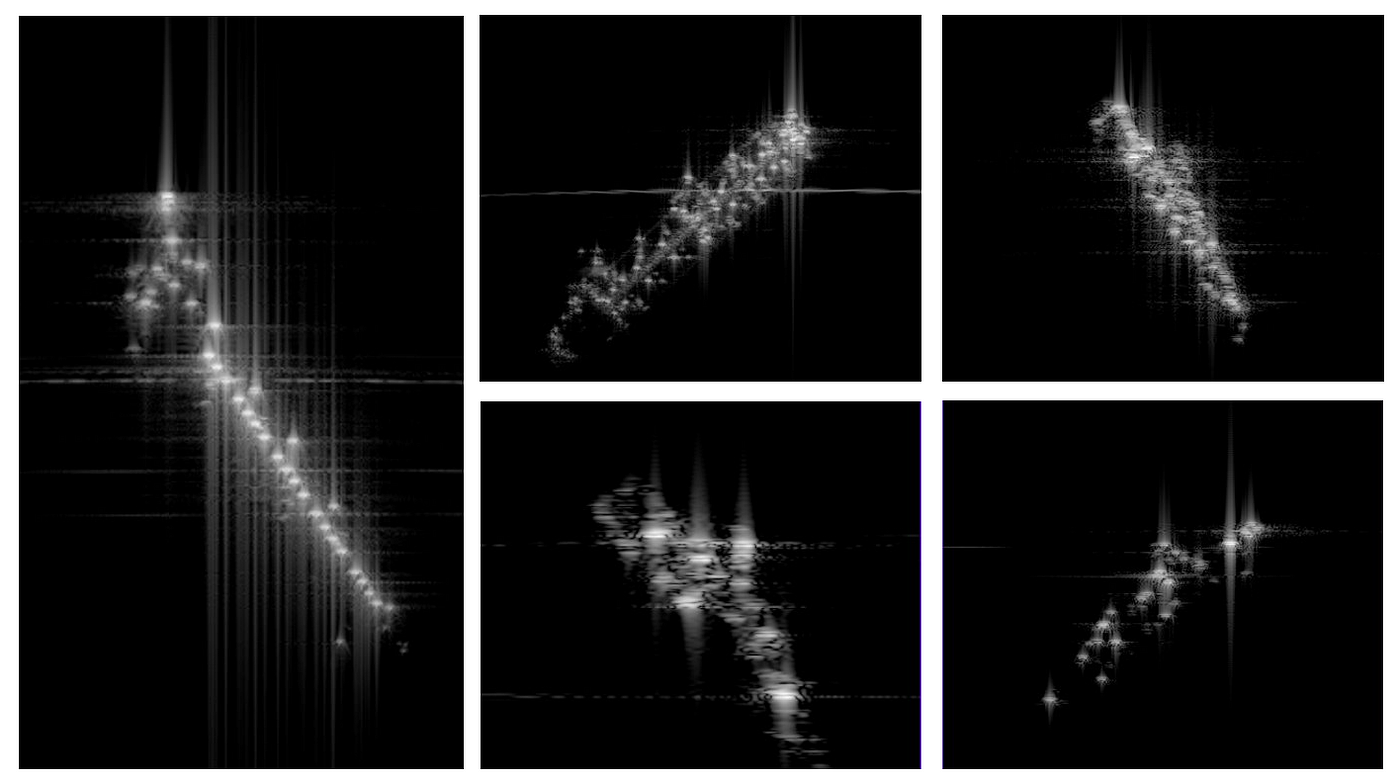
Simulated SAR captures of marine vessels using Rendered.ai. Rendered.ai is a PaaS for synthetic data generation for AI training and validation.
Tl;DR: Synthetic Aperture Radar (SAR) is changing how we view our planet. With the growing interest in developing artificial intelligence (AI) algorithms for object recognition and classification, we hear from customers who want to access SAR datasets to test and train algorithms. Rendered.ai is publicly sharing a demo of our capability to generate SAR imagery. To try it for yourself, sign up for the Rendered.ai platform and input the content code ‘SARDEMO’ or contact us at sales@rendered.ai
Introduction
I won’t talk about what Synthetic Aperture Radar is or why it’s fantastic. NASA, Esri, and Wikipedia have plenty of material covering SAR concepts. I want to talk about what is happening now with SAR, why you should care, and why building a simulation is critical to getting the most out of measured SAR images.
There are 50+ commercial SAR satellites in the sky today, and there will be over 100 before the end of the decade. Soon, there will be sub-hour revisit times for much of the Earth with image resolutions better than 1m², 24 hours a day, and under all atmospheric conditions (including clouds and smoke). In the future, the amount of SAR data available to be analyzed will be enormous, and the amount of potential information to glean from those images will only be limited by our analytics and AI algorithms.
What does this mean?
Electro-Optic (EO) imagery, imagery of the visible light spectrum, can only be captured during the day without cloud cover. With only half of the Earth illuminated at any time and cloud cover typically approaching 67%, getting images for time-critical or persistent observation is difficult. SAR provides the capability to capture imagery of any location on the planet independent of environmental and lighting conditions.
Here are just a few industries that SAR will have an impact on:
Agriculture
Farmers are always trying to optimize the value of their crops, whether by increasing yield, providing just enough water, or managing insects to keep crops growing safely. However, monitoring thousands of acres requires significant investment and infrastructure. Satellite SAR mitigates the need for that investment and infrastructure, as images can be purchased for about $1000 that cover a 30×50 km footprint or larger.
From those images, important information can be extracted, such as soil moisture levels, crop growth, and other changes in vegetation. A study even attempted to predict crop yields which would be helpful for farmers and commodities traders as predicting crop production numbers could be an early indicator for the commodities market.
Border Security
SAR’s ability to provide persistent satellite surveillance under all weather conditions makes it ideal for monitoring borders stretching for thousands of miles. Ground-based infrastructure is expensive and requires maintenance. However, SAR can provide images at regular intervals so border control officials can be cued to specific locations using object detection instead of having to patrol the border, allowing for better use of their time and resources.
Defense & Intelligence
SAR has most recently played a huge role in the war in Ukraine by providing daily status of the entire warzone. It was even used to identify that a conflict was about to break out when Capella Space provided a SAR image to a professor at the Middlebury Institute of International Studies (MIIS) on February 23rd, 2022, the day before Russia invaded, showing tanks and other vehicles lined up and ready to invade.
How do you get information from those images?
In the same way that AI/ML algorithms have superseded every other method developed to identify objects in an image, the same techniques can be used on images created with SAR. A significant requirement to successfully implement AI/ML is having a large and diverse set of annotated training data. This is where the value proposition for SAR simulation makes sense. While a single SAR image may cost $1000 from a provider, it only costs pennies to simulate, and the diversity of images is unlimited. For example, the short video below is of a cargo ship at 30 degree look angle with the vessel rotated every two degrees. Creating this from a measured dataset would be prohibitively expensive and impossible due to all the geometries required to be captured.
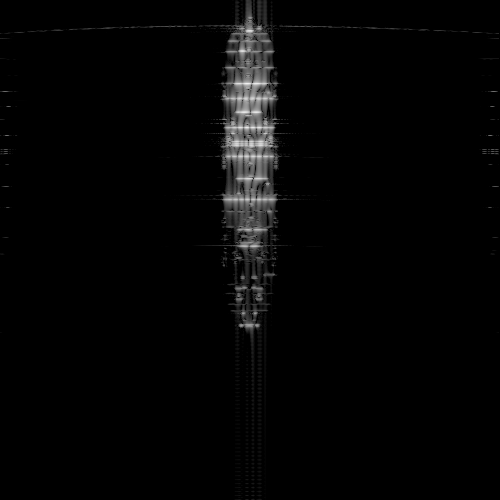
Example of a simulated SAR capture of a cargo ship created with Rendered.ai.
SAR Simulation for Data Scientists
A simulation provides several valuable capabilities:
- The cost to generate images is reduced to compute time required to perform the simulation… and many simulations can be run to create large datasets for AI training.
- Pixel-level annotation/labeling is easy to generate because the simulation knows the underlying truth of where targets are in the scene.
- Creating training images for a new target is as easy as adding a new 3D model.
Using a simulation solves significant problems in creating an effective AI/ML algorithm. However, it also introduces some new questions.
- How accurate of a 3D model is required?
- Does clutter need to be modeled? What type of backgrounds: ocean, sand, dirt, grass?
- Do atmospheric impacts need to be included? Radars can see through clouds and smoke, but the signal changes when it passes through those things. That change will impact the resulting image.
- What other conditions must be addressed for the simulation to generate enough realism?
Each question adds a layer of fidelity to the simulation, but all of them might not be necessary. Each level of fidelity is a tool in the toolbox that the data scientist can use to understand better what is needed to train an AI/ML model to accurately and reliably classify an object. The image below shows this iterative process includes data scientists and synthetic data engineers.
Conclusion
Synthetic Aperture Radar has the potential to revolutionize many industries by making information available that was either too expensive or impossible to get before. Of course, extracting that information will still take effort, but the SAR simulator at Rendered.ai can help simplify the creation of your training data.
Get started generating synthetic aperture radar (SAR) AI training data!
We have released a demo of the capabilities of our SAR synthetic data channel under the content code ‘SARDEMO’.
- If you are new to the platform, first request access, then input the content code on the registration page once you receive a link.
- If you already have an account, enter this code in the field labeled ‘Content Code’ when setting up a new workspace.
- Follow this link for more information on content codes, along with complete platform documentation, follow this link.
If you’re interested in learning more about how synthetic data improves the training and validation process in AI and ML models and you’d like a technical deep dive into our SAR channel:
- Contact us at sales@rendered.ai
Credits
Thank you to Capella Space for publishing some of the content referenced in this article.