
TL;DR: After one year at Rendered.ai, I’m seeing common questions from customers that indicate awareness of synthetic data is growing, many customers are trying to create synthetic data for themselves, and customers are starting to seek sustainable synthetic data capability whether through staffing or through seeking business models and implementation patterns that work with their enterprise AI pipelines.
I just passed my one-year anniversary with Rendered.ai and it’s been a whirlwind and a thrill! I have never looked back. After being in so many emerging product and technology efforts over my career, I can’t imagine not being in this type of role where I get to directly contribute to the culture, product, and growth of a company that is helping to shape a high-impact, rapidly growing market.
At Rendered.ai, we’ve been running full speed, typical #startuplife, so I’ve been a bit quieter than usual. Now, because we’re growing our team and I’m able to focus on some of the core activities I’ve always enjoyed, such as sharing about lessons learned and successes.
One of my favorite activities is engaging with customers, partners, and analysts and distilling their questions and feedback down to essential questions and answers that can help others navigate the complexity and nuance of new tech and opportunity. We’ve run into two major categories of questions and comments that I’ve identified here, basic questions about what we do and questions or comments about the value of synthetic data and approaches to training AI. The following questions aren’t exhaustive, but reveal insights on many of our initial conversations with AI community members exploring synthetic data.
So… what does Rendered.ai do?
You’ll typically hear us saying that Rendered.ai is a Platform as a Service for synthetic data that enables data scientists to overcome the costs and challenges of acquiring and using real data for training machine learning and artificial intelligence systems.
What that really means is, we help our customers to achieve better control over their AI so that they can more quickly, cheaply, and accurately solve real business problems.
AI is driven by data. The world is awash in data, however, so much of that data is unlabeled, biased, or irrelevant for specific users’ problems. Dr. Nathan Kundtz, our CEO, realized years ago that a way to overcome this problem is to imagine, and then intentionally create, data that can be used to train AI to achieve specific results. Hence our tagline:
Imagine data that matters!
What is synthetic data?
Synthetic data is engineered data that is intentionally designed to have characteristics that address a specific problem with real sensor data or with an AI training or validation process.
Real-world data typically fall into two categories, structured and unstructured, indicating whether the data have predefined, known schema or ‘structure.’ Data scientists categorize form-based text data, DNA, and stock prices as structured data, for example.
Imagery, lidar, video, and other pixel or voxel-based data are considered unstructured data. You may be able to look at a couple of pixels and see a child’s toy, but there is no intrinsic information in the pixels themselves that classify those specific pixels as a toy, for example. Unstructured datasets associated with physical sensor-based data capture are used in computer vision (CV) and machine vision (MV) applications, which are highly dependent on the specific values and patterns of pixels (or voxels or points) contained in sensor-derived datasets.
Techniques for the creation of synthetic data fall into similar categories, with some synthetic data attempting to replicate structured data and other synthetic data built for unstructured data. Generally, Rendered.ai focuses on enabling customers to simulate physics-based data for the purposes of training CV or MV algorithms that will process unstructured data including satellite imagery, Synthetic Aperture Radar (SAR), and pretty much any type of imagery, video, or other pixel-like information.
What do we mean by physics-based synthetic data?
While there are many different methods to obtain unstructured data, essentially any type of pixel output, Rendered.ai’s customers predominantly focus on processing data such as camera imagery, SAR, x-ray, or other physically created sensor data. We often refer to sensor-captured data as physics-based. Simulating physics-based data can involve different steps with the ultimate point being to simulate pixels as if they were created by a physical system. We don’t have to simulate a real system down to the atomic movement. We need to help customers create synthetic imagery as if it was created by such physical systems.
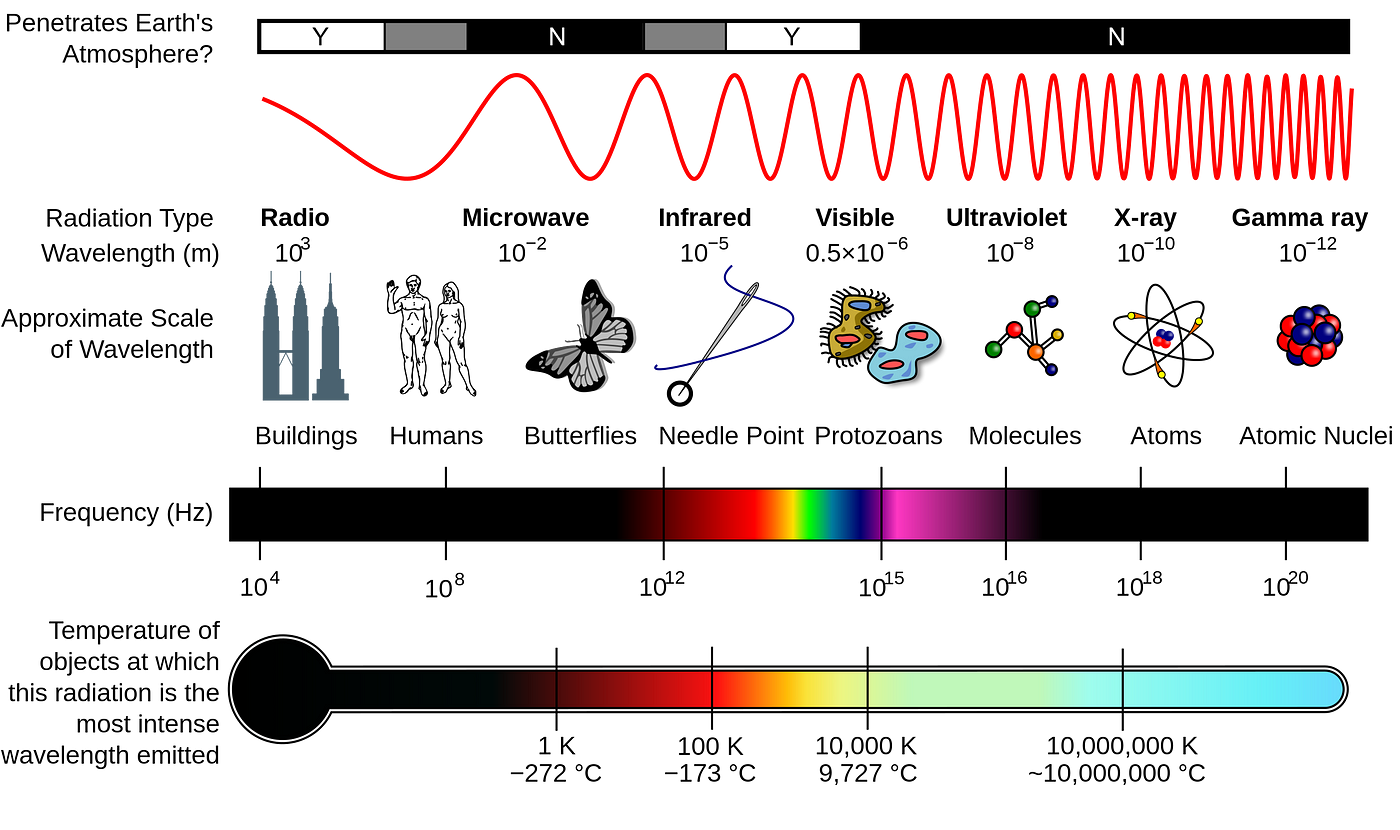
To simulate physical data capture, we help our customers build channels, or synthetic data applications, that take advantage of diverse tools including some of the following:
· Creating a 3D ‘digital twin’ of a sensor collection scenario — This could include digitally modeling electromagnetic radiation propagation properties, sensor characteristics, characteristics of the platform upon which the sensor is mounted, and much more
· Simulating capture issues through post-processing effects — In cases of lens distortion, dirty equipment, inconsistent operation, or even some environmental issues such as mist or heat distortion, these effects can often be created through post-processing simulated imagery from the digital sensor simulation.
· Domain adaptation — In other cases, there may be intrinsic properties of a real dataset that are ubiquitous but hard to simulate. In some of these cases, we are able to train a CycleGAN using real imagery and then use the CycleGAN to post-process synthetic imagery, ending up with a dataset that appears more like the real thing to AI.
Every synthetic data project starts out with a hypothesis of what the ideal data characteristics are that would be required to train or validate AI. In addition to these techniques, we also provide tools for comparing datasets to begin to see how close a synthetic dataset is to the real thing.
Does Rendered.ai sell datasets?
We do not sell datasets.
After working with a wide range of customers, real CV applications never need just one dataset. It’s conceivable that we could provide a dataset or two for testing, but invariably what we could create on our own would not solve a customer’s actual problem. Our preference is not to create datasets at all, but to help our customers solve their initial problem and then become self-sufficient on the platform, configuring and regenerating datasets as they need them.
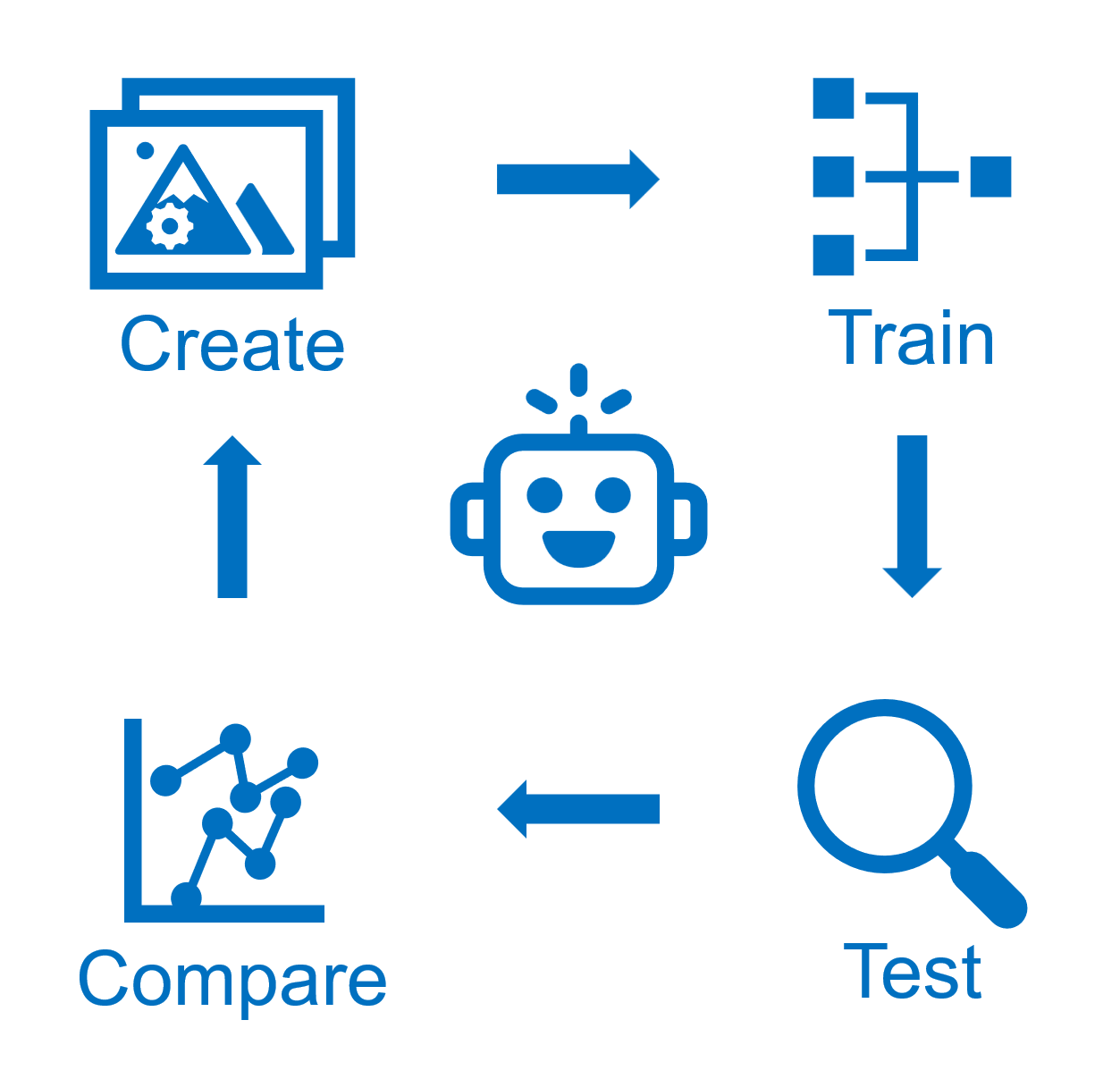
Why do customers engage with Rendered.ai?
Customers come to us because we provide a platform that can accommodate a broad range of simulated sensor output, build configurability into synthetic data generation to create diverse datasets for training and validation, and because implementing a synthetic data application on top of Rendered.ai enables the customer to capture and preserve institutional knowledge about their specific sensor, data type, or even analysis problem.
In other words, customers work with us because reliable, sustainable access to synthetic data helps them solve their AI training problems rapidly and with the ability to deeply understand what characteristics of their data contributed to specific outcomes with their AI. As AI becomes more integrated with all manner of business operations across diverse industries, we anticipate the need for synthetic data as an enterprise capability to grow continuously.
Common customer challenges
Discovering, designing, and creating synthetic data to train and validate AI is not new, but it’s also not a common aspect of enterprise AI programs. In some cases, our customers have tried to grow their own synthetic data applications and met mixed results. In other cases, customers have purchased a dataset and been less than happy with training outcomes. These issues don’t necessarily mean that synthetic data won’t work. Often, we find that customers encountered common pitfalls when implementing a new technology for the first time.
We have tried synthetic data and it didn’t work
Of those customers who have heard of synthetic data, we encounter a reasonable number who have tried to create or work with synthetic data in some way. Most of these came away with mixed results. Some of the typical patterns we see are customers who have:
· Paid for one-time datasets
· Experimented with a simulator or game engine for a single project
· Attempted to modify existing real sensor data for a project or application
In most cases in which a customer has tried synthetic data, the customer approached the effort as a one-off training experiment. We find that this is typical when customers are focused on traditional data science workflows that rely on real data. They are constrained to use only the data that they have… which is not a problem with the effectively infinite variety of synthetic data that can be created!
I’ve seen synthetic data and it didn’t look realistic
The outcomes of training an AI for CV applications are extremely sensitive to the pixel values and distributions in training data. Subtle variations in pattern, sequence, or intensity values across training datasets that are inconsequential to human interpretation can lead to significantly different AI performance in production applications.
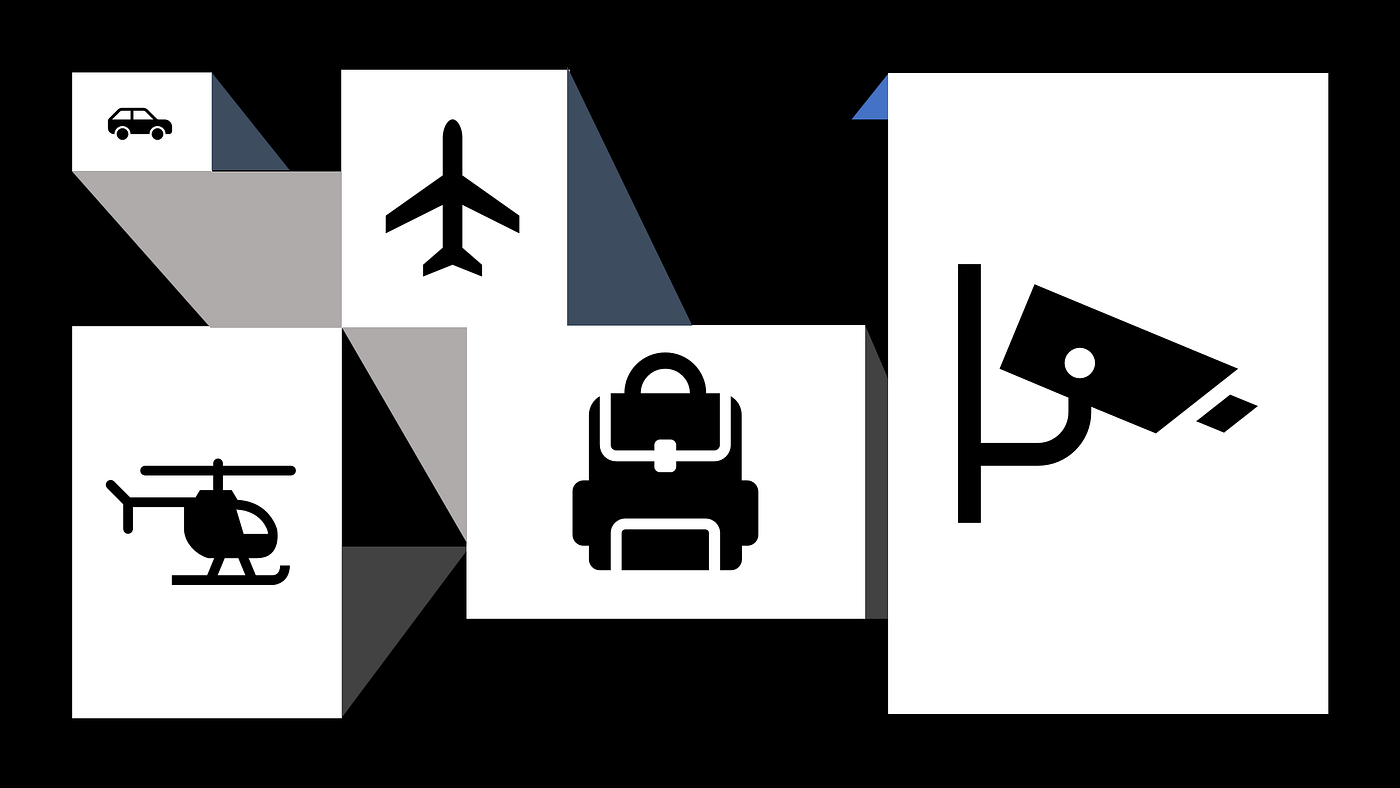
If synthetic images are too cartoonish, have unrealistic patterns, or otherwise lack the properties of real sensor images, then an AI trained from those images may perform poorly or even fail to detect or classify critical features in a dataset. A human may not even notice a gap between real and synthetic datasets, but an algorithm may end up being influenced strongly by issues with generated imagery.
We have seen repeatedly that successful AI training with synthetic data requires iteration and the application of multiple techniques to achieve data that behaves as if it is real data to AI. Some of the techniques that we enable customers to apply include:
· Control over scene composition, object distribution, and object or classified surface frequency in simulated imagery
· Application of environmental and post-processing effects during image generation such as adding weather, dirt, or lens distortion
· Domain adaptation using CycleGANs trained on real sensor data to apply effects to datasets that add subtle characteristics that change the characteristics of synthetic data to appear more like the training set
Ultimately, it may take multiple techniques to generate synthetic data that behaves like real data. It also helps to have tools to compare datasets so that users can investigate if changes they are making are causing synthetic data to behave more like real data. For that purpose, we offer a built-in UMAP domain reduction analysis tool and we will be adding more dataset investigation tools over time.
I don’t have 3D modeling and simulation skills
One of the most problematic issues in a tight labor market is that many of our customers may not have the in-house skills to create synthetic data. For these customers, we offer the following:
· Our Enterprise Subscription level includes Enhanced Support Credits that covers onboarding to get customers to the point of generating their first valid datasets
· Through professional services, our expert consultants will act as the Synthetic Data Engineers for our customers
· We also have technical and educational content to help customers get started quickly on our platform
You can learn more about this in this blog on Synthetic Data Engineers!
The TCO of a synthetic data stack is too high
When an organization attempting to improve CV applications looks at the total investment to run simulations in the cloud and repeatedly generate synthetic data as an ongoing capability, they are often concerned about maintaining another enterprise technology stack along and having the right staff who are knowledgeable about a rapidly evolving technology landscape. In the synthetic data space, it seems like new advancements in cloud instances with GPUs, new game engines, and new AI techniques for generating synthetic imagery are coming out on a daily basis.
For these customers, Rendered.ai offers a single portal into synthetic data generation capability that will be continuously expanded upon within a consistent subscription model. Our Enterprise Subscription tier is a fraction of the investment that a customer might need to make in staff and infrastructure to support a home-grown capability. Rendered.ai is also now available on the AWS Marketplace for customers who want to fold access to synthetic data capability into their AWS enterprise purchasing.
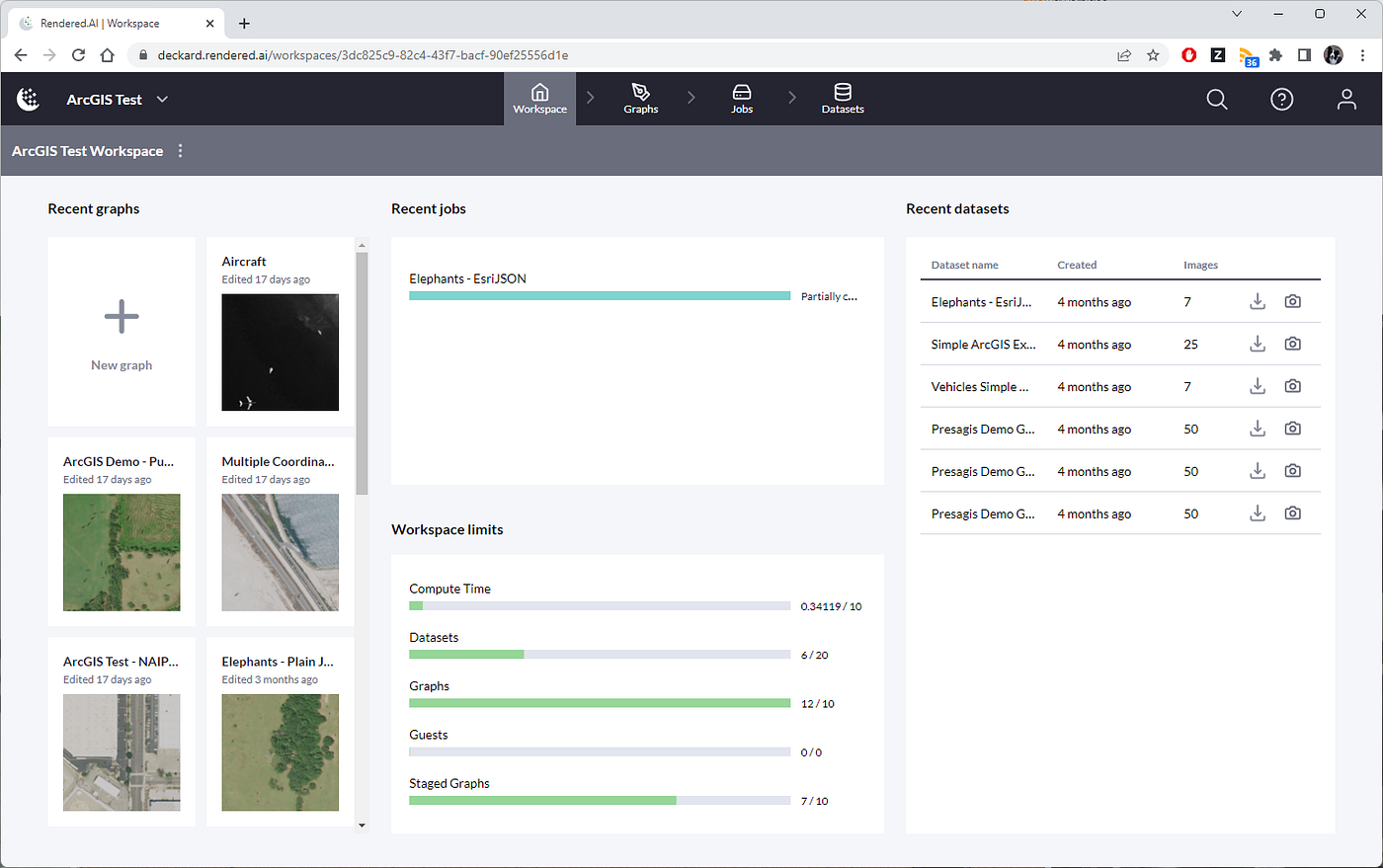
Find out more… or generate a dataset for yourself!
There are multiple paths to find out more about synthetic data and Rendered.ai. Our support documentation is open. Anyone can sign up to try out our platform. We also publish numerous blogs, interviews, and videos covering the value of synthetic data and how to use our platform.
Follow these links to find out more:
· Sign up to get started generating your own synthetic data on the Rendered.ai platform
· Check out our learning path on our support site
· Here’s an overview video of the platform
And if you have any other questions, you can always contact us here!