Ansys and Rendered.ai, a member of the Ansys Developer Partner Program, have combined forces to bring faster simulation of large environments to remote sensing professionals using computer vision to analyze Synthetic Aperture Radar (SAR) data.
Why SAR?
Remote sensing SAR data has been in limited use for decades. However, the emergence of commercial satellite operators with greater capacity for collection and new types of SAR collection has increased the geospatial intelligence community’s effort to incorporate SAR into analysis workflows. SAR can be collected through cloud cover on the night side of the planet, and it can be useful for identifying objects with different material types. So, there’s tremendous interest in using SAR for around-the-clock situational awareness of natural disasters, conflict zones, and changing environments such as large mines and construction areas.
One of the challenges of working with SAR is that a SAR image is not a black-and-white or color photograph. Each pixel in a SAR image represents an interpolation of values of reflected electromagnetic waves from multiple points in a scene. Non-visible light sensor data, such as SAR, typically requires additional expertise for interpretation, which can become expensive when labeling large datasets. The technical challenges of obtaining and interpreting SAR data make it ideal for supporting physically modeled synthetic data, such as what is possible with Perceive EM and Rendered.ai.
Perceive EM in the Rendered.ai PaaS
The collaboration focuses on using Rendered.ai’s open source SDK to create synthetic data channels with PyAnsys by providing a wrapper around Ansys’ Perceive EM. Through this combination, a customer can use Rendered.ai’s synthetic data generation platform to create large datasets of SAR images using Perceive EM simulation software in the cloud. Computer vision teams will be able to use this integration to create massive amounts of data to train AI/ML systems to process SAR data.
Customers deploying the combined offering will gain two key advantages:
- Access to Rendered.ai’s drag-and-drop configuration interface to design scenes and scenarios with physically accurate material properties
- The ability to use cloud processing to rapidly synthesize an unlimited number of configurable SAR datasets based on those scenes and scenarios
Are you interested in learning how this collaboration can improve your SAR workflows? Request a consultation to learn about pricing and how to deploy this combined offering.
A standardized offering for broader usage will also be released at a later date, so stay tuned!
Real-World Example: Synthetic SAR On Demand
To showcase the power of using the Perceive EM simulator and the Rendered.ai platform for synthetic SAR data generation on demand, we wanted to show that we could generate imagery for a large, real-world area.
One of our experts, Jeffrey Ian Wilson, Rendered.ai’s 3D Technical Director, obtained permission to fly drone imagery for a decommissioned air base in California. Jeff processed the imagery through a reality modeling workflow to generate a textured mesh of the area that could be used in visualization and simulation projects, such as our collaboration with the Perceive EM team.
Jeff’s aerial 3D scan captured 3cm2 nadir imagery. The drone imagery was merged with USGS aerial lidar data and satellite imagery with RTK/GNSS ground control, resulting in 5-10 mm spatial accuracy for the whole dataset.
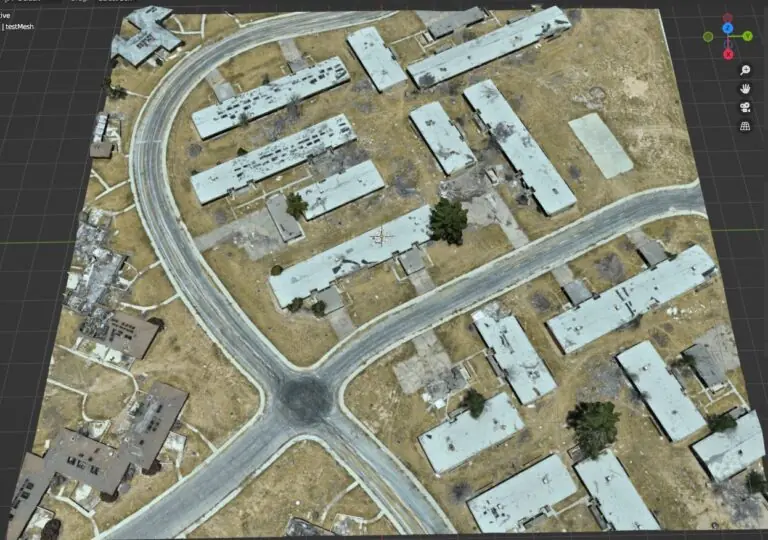
Jeff then worked with Rendered.ai colleagues to combine the 3D mesh of the air base with 3D models of military tanks within the Rendered.ai platform to create a scene.
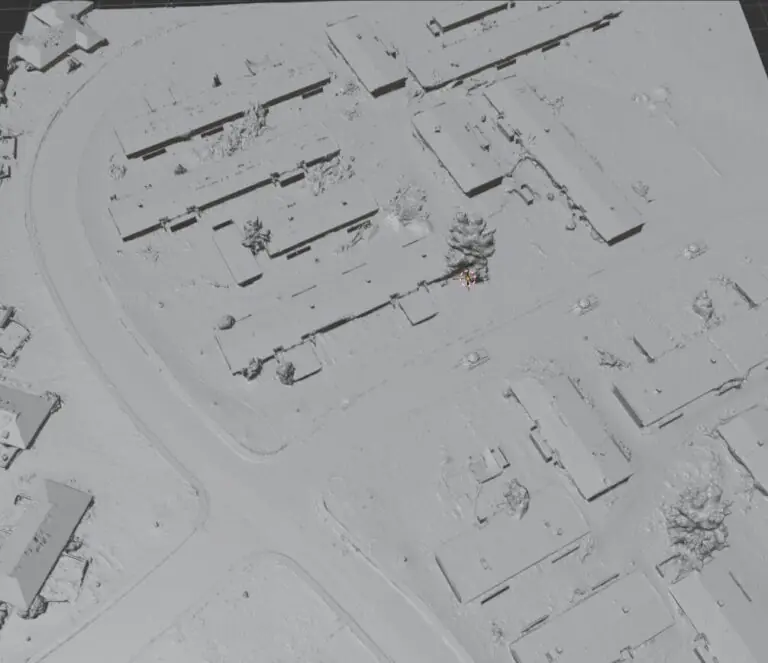
The combined scene was processed through the Perceive EM simulator to create SAR imagery. Using this combination of data and simulator, each simulated image of military tanks driving through the air base took less than a minute to generate.
Note that RGB textures were ignored for this simulation as they are unnecessary for SAR. A uniform material type was applied to the reality mesh for this simulation. The mesh, however, could also have been subdivided, and more refined material properties could have been applied to different groups of triangles.
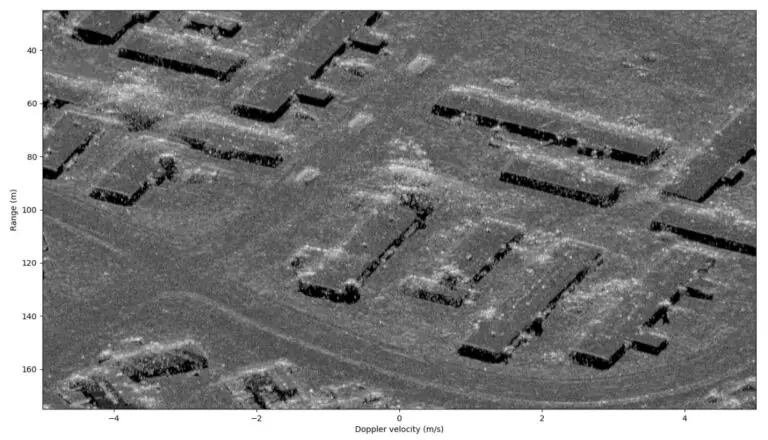
Once 3D assets and a simulator are available in the Rendered.ai platform, they can be used in a channel, our term for a synthetic data application that runs on our PaaS. A channel can be used to create a nearly unlimited range of data capture scenarios. For example, the tanks used in this simulation could be swapped out for fueling trucks or other objects. Even the numbers and positions of objects of interest can be varied.
In addition to having access to large datasets through cloud simulation, the data becomes even more valuable for algorithm training because it’s possible to generate automated annotation, classifying and identifying the location of each instance of a vehicle or other asset in each image.
Learn More
This is just one example of how the Ansys Perceive EM simulator, PyAnsys, and the Rendered.ai platform can be used together to quickly generate fully labeled simulated imagery in large quantities for training AI and ML detectors. The Rendered.ai platform enables computer vision engineers to build and share configurations for unlimited synthetic data generation to train computer vision algorithms to interpret new and hard-to-interpret data sources, such as remote sensing SAR imagery.
If you would like to learn more about simulating SAR data with Perceive EM in the Rendered.ai platform, watch our “SAR Simulation with Reality Capture & Ansys” webinar on demand now!